U.S. President Donald Trump announced a private-sector investment of up to $500 billion to build artificial intelligence (AI) infrastructure across the United States. Dubbed “The Stargate Project,” this massive initiative is expected to accelerate America’s AI capabilities, create hundreds of thousands of jobs, and bolster national…
New general law governs fracture energy of networks across materials and length scales
Materials like car tires, human tissues, and spider webs are diverse in composition, but all contain networks of interconnected strands. A long-standing question about the durability of these materials asks: What is the energy required to fracture these diverse networks? A recently published paper by MIT researchers offers new insights.
“Our findings reveal a simple, general law that governs the fracture energy of networks across various materials and length scales,” says Xuanhe Zhao, the Uncas and Helen Whitaker Professor and professor of mechanical engineering and civil and environmental engineering at MIT. “This discovery has significant implications for the design of new materials, structures, and metamaterials, allowing for the creation of systems that are incredibly tough, soft, and stretchable.”
Despite an established understanding of the importance of failure resistance in design of such networks, no existing physical model effectively linked strand mechanics and connectivity to predict bulk fracture — until now. This new research reveals a universal scaling law that bridges length scales and makes it possible to predict the intrinsic fracture energy of diverse networks.
“This theory helps us predict how much energy it takes to break these networks by advancing a crack,” says graduate student Chase Hartquist, one of the paper’s lead authors. “It turns out that you can design tougher versions of these materials by making the strands longer, more stretchable, or resistant to higher forces before breaking.”
To validate their results, the team 3D-printed a giant, stretchable network, allowing them to demonstrate fracture properties in practice. They found that despite the differences in the networks, they all followed a simple and predictable rule. Beyond the changes to the strands themselves, a network can also be toughened by connecting the strands into larger loops.
“By adjusting these properties, car tires could last longer, tissues could better resist injury, and spider webs could become more durable,” says Hartquist.
Shu Wang, a postdoc in Zhao’s lab and fellow lead author of the paper, called the research findings “an extremely fulfilling moment … it meant that the same rules could be applied to describe a wide variety of materials, making it easier to design the best material for a given situation.”
The researchers explain that this work represents progress in an exciting and emerging field called “architected materials,” where the structure within the material itself gives it unique properties. They say the discovery sheds light on how to make these materials even tougher, by focusing on designing the segments within the architecture stronger and more stretchable. The strategy is adaptable for materials across fields and can be applied to improve durability of soft robotic actuators, enhance the toughness of engineered tissues, or even create resilient lattices for aerospace technology.
Their open-access paper, “Scaling Law for Intrinsic Fracture Energy of Diverse Stretchable Networks,” is available now in Physical Review X, a leading journal in interdisciplinary physics.
“Forever grateful for MIT Open Learning for making knowledge accessible and fostering a network of curious minds”
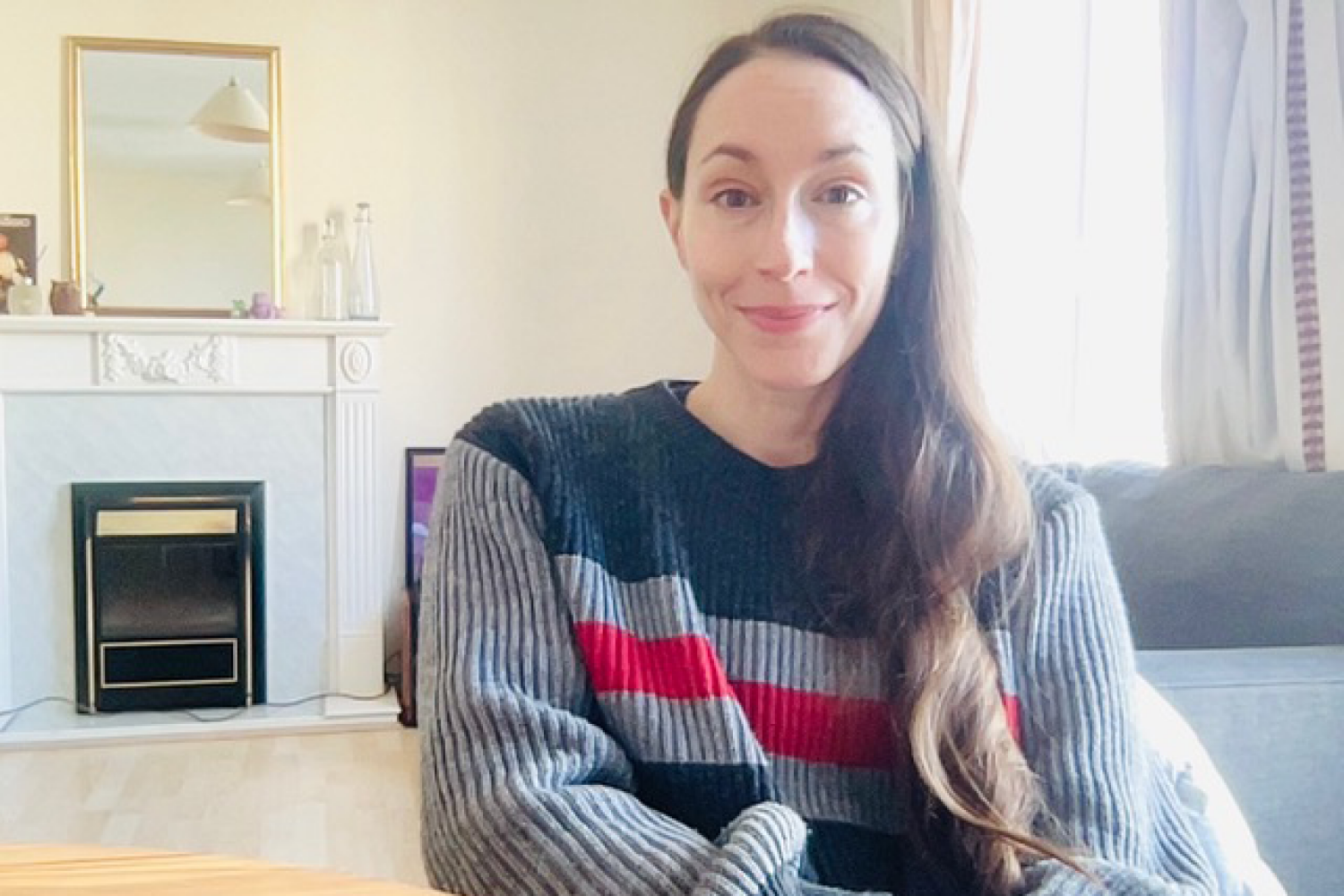
Bia Adams, a London-based neuropsychologist, former professional ballet dancer, and MIT Open Learning learner, has built her career across decades of diverse, interconnected experiences and an emphasis on lifelong learning. She earned her bachelor’s degree in clinical and behavioral psychology, and then worked as a psychologist and therapist for several years before taking a sabbatical in her late 20s to study at the London Contemporary Dance School and The Royal Ballet — fulfilling a long-time dream.
“In hindsight, I think what drew me most to ballet was not so much the form itself,” says Adams, “but more of a subconscious desire to make sense of my body moving through space and time, my emotions and motivations — all within a discipline that is rigorous, meticulous, and routine-based. It’s an endeavor to make sense of the world and myself.”
After acquiring some dance-related injuries, Adams returned to psychology. She completed an online certificate program specializing in medical neuroscience via Duke University, focusing on how pathology arises out of the way the brain computes information and generates behavior.
In addition to her clinical practice, she has also worked at a data science and AI consultancy for neural network research.
In 2022, in search of new things to learn and apply to both her work and personal life, Adams discovered MIT OpenCourseWare within MIT Open Learning. She was drawn to class 8.04 (Quantum Physics I), which specifically focuses on quantum mechanics, as she was hoping to finally gain some understanding of complex topics that she had tried to teach herself in the past with limited success. She credits the course’s lectures, taught by Allan Adams (physicist and principal investigator of the MIT Future Ocean Lab), with finally making these challenging topics approachable.
“I still talk to my friends at length about exciting moments in these lectures,” says Adams. “After the first class, I was hooked.”
Adams’s journey through MIT Open Learning’s educational resources quickly led to a deeper interest in computational neuroscience. She learned how to use tools from mathematics and computer science to better understand the brain, nervous system, and behavior.
She says she gained many new insights from class 6.034 (Artificial Intelligence), particularly in watching the late Professor Patrick Winston’s lectures. She appreciated learning more about the cognitive psychology aspect of AI, including how pioneers in the field looked at how the brain processes information and aimed to build programs that could solve problems. She further enhanced her understanding of AI with the Minds and Machines course on MITx Online, part of Open Learning.
Adams is now in the process of completing Introduction to Computer Science and Programming Using Python, taught by John Guttag; Eric Grimson, former interim vice president for Open Learning; and Ana Bell.
“I am multilingual, and I think the way my brain processes code is similar to the way computers code,” says Adams. “I find learning to code similar to learning a foreign language: both exhilarating and intimidating. Learning the rules, deciphering the syntax, and building my own world through code is one of the most fascinating challenges of my life.”
Adams is also pursuing a master’s degree at Duke and the University College of London, focusing on the neurobiology of sleep and looking particularly at how the biochemistry of the brain can affect this critical function. As a complement to this research, she is currently exploring class 9.40 (Introduction to Neural Computation), taught by Michale Fee and Daniel Zysman, which introduces quantitative approaches to understanding brain and cognitive functions and neurons and covers foundational quantitative tools of data analysis in neuroscience.
In addition to the courses related more directly to her field, MIT Open Learning also provided Adams an opportunity to explore other academic areas. She delved into philosophy for the first time, taking Paradox and Infinity, taught by Professor Agustín Rayo, the Kenan Sahin Dean of the MIT School of Humanities, Arts, and Social Sciences, and Digital Learning Lab Fellow David Balcarras, which looks at the intersection of philosophy and mathematics. She also was able to explore in more depth immunology, which had always been of great interest to her, through Professor Adam Martin’s lectures on this topic in class 7.016 (Introductory Biology).
“I am forever grateful for MIT Open Learning,” says Adams, “for making knowledge accessible and fostering a network of curious minds, all striving to share, expand, and apply this knowledge for the greater good.”
For MIT-WHOI Joint Program student Faith Brooks, the sky’s the limit
Faith Brooks, a graduate student in the MIT-WHOI Joint Program, has had a clear dream since the age of 4: to become a pilot.
“At around 8 years old, my neighbor knew I wanted to fly and showed me pictures of her dad landing a jet on an aircraft carrier, and I was immediately captivated,” says Brooks. Further inspired by her grandfather’s experience in the U.S. Navy (USN), and owing to a lifelong fascination with aviation, she knew nothing would stand in her way.
Brooks explored several different paths to becoming a pilot, but she says one conversation with her longtime mentor, Capt. Matt Skone, USN (Ret.), changed the trajectory of her life.
“He asked if I had heard of the Naval Academy,” she recalls. “At the time, I hadn’t … I immediately knew that that was where I wanted to go, and everything else I learned about United States Naval Academy (USNA) reinforced that for me.”
In her “firstie” (senior) year at the USNA, Brooks was selected to go to Pensacola, Florida, and train to become a naval pilot as a student naval aviator, taking her one step closer to her dream. The USNA also helped guide her path to MIT. Her journey to joining the MIT-WHOI Joint Program began with the USNA’s professional knowledge curriculum, where she read about retired Capt. Wendy Lawrence SM ’88, a naval aviator and astronaut.
“Reading her bio prompted me to look into the program, and it sounded like the perfect program for me — where else could you get a better education in ocean engineering than MIT and Woods Hole Oceanographic Institution [WHOI]?”
In the MIT-WHOI Joint Program, Brooks is researching the impact of coastal pond breaching on preventing and mitigating harmful algal blooms. Her work focuses on the biannual mechanical breaching of Nantucket’s Sesachacha Pond to the ocean and the resultant impact on the pond’s water quality. This practice aims to improve water quality and mitigate harmful algal blooms (HABs), especially in summer.
Breaching in coastal ponds is a process that was initially used to enhance salinity for herring and shellfish habitats, but has since shifted to address water quality concerns. Traditionally, an excavator creates a breach in the pond, which naturally closes within one to five days, influenced by sediment transport and weather conditions. High winds and waves can accelerate sediment movement, limiting ocean water exchange and potentially increasing eutrophication, where excessive nutrients lead to dense plant growth and depletion of oxygen. In brackish water environments, harmful algal blooms are often driven by elevated nitrogen levels and higher temperatures, with higher nitrogen concentrating leading to more frequent and severe blooms as temperatures rise.
The Nantucket Natural Resources Department (NRD) has been collaborating with local homeowners to investigate the pond breaching process. Existing data are mainly anecdotal evidence and NRD’s monthly sampling since 2022, which has not shown the expected decrease in eutrophication. Brooks’ research will focus on data before, during, and after the breach at two pond sites to assess water changes to evaluate its effectiveness in improving water quality.
When Brooks isn’t knee-deep in the waters of the Sesachacha or training with her MIT Triathlon team, she takes additional opportunities to further her education. Last year, Brooks participated in the MIT-Portugal Marine Robotics Summer School in Faial, Azores, in Portugal, and immersed herself in a combination of a hands-on design projects and lectures on a variety of topics related to oceanography, engineering, and marine robotics.
“My favorite part of the program was how interdisciplinary it was. We had a combination of mechanical engineers, electrical engineers, computer scientists, marine biologists, and oceanographers, and we had teams that included each of these specialties,” she says. “Our project involved designing a lander equipped with an underwater camera connected to a surface buoy that would transmit the footage. Having worked in mostly just engineering teams previously, it was a great experience to work with a more diverse group and I gained a much better understanding of how to design instruments and systems in accordance with what the marine biologists need.”
Brooks also earned her Part 107 Small Unmanned Aircraft System (UAS) license to operate the lab’s drone with a multispectral camera for her upcoming fieldwork. When she graduates from the MIT-WHOI Joint Program next September, she’ll report to the Naval Aviation Schools Command in Pensacola, Florida, to begin flight training.
While she says she’ll miss Boston’s charm and history, as well as the Shining Sea Bikeway on crisp fall days in Woods Hole, Brooks is looking forward to putting her uniform back on, and starting her naval career and flight school. The time Brooks has spent at MIT will support her in these future endeavors. She advises others interested in a similar path to focus on research within their areas of interest.
“The biggest lesson that I’ve learned from both research theses is that any research project will change over time, and it’s often a good idea to take a step back and look at how your work fits into the larger picture,” she says. “I couldn’t recommend doing research more; it’s such a great opportunity to dig into something that you’re interested in, and is also very fulfilling.”
It’s Time to Pass the AI Baton From Software to Hardware
It’s unlikely that we’re going to encounter any technology more consequential and important than AI in our lifetimes. The presence of artificial intelligence has already altered the human experience and how technology can reshape our lives, and its trajectory of impact is only getting wider. With…
How Google Cloud’s Automotive AI Agent is Transforming In-Car Experience with Mercedes-Benz
The relationship between artificial intelligence (AI) and automobiles has been evolving for decades, transitioning from basic automation to today’s advanced self-driving technologies. This evolution has entered a new phase with the advent of AI agents that not only assist with driving but also transform how drivers…
Grace Yee, Senior Director of Ethical Innovation (AI Ethics and Accessibility) at Adobe – Interview Series
Grace Yee is the Senior Director of Ethical Innovation (AI Ethics and Accessibility) at Adobe, driving global, organization-wide work around ethics and developing processes, tools, trainings, and other resources to help ensure that Adobe’s industry-leading AI innovations continually evolve in line with Adobe’s core values and…
Bryan Reimer named to FAA Rulemaking Committee
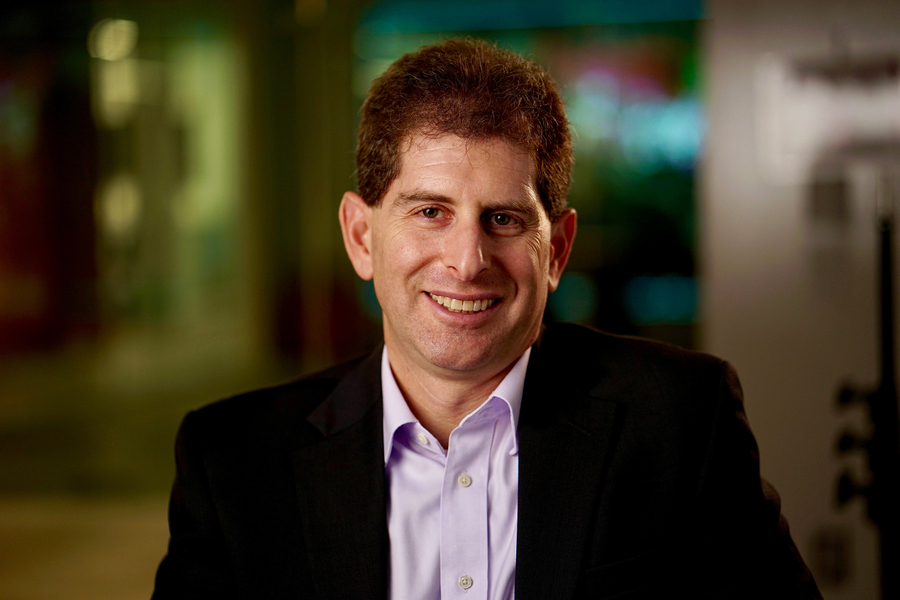
Bryan Reimer, a research scientist at the MIT Center for Transportation and Logistics (CTL), and the founder and co-leader of the Advanced Vehicle Technology Consortium and the Human Factors Evaluator for Automotive Demand Consortium in the MIT AgeLab, has been appointed to the Task Force on Human Factors in Aviation Safety Aviation Rulemaking Committee (HF Task Force ARC). The HF Task Force ARC will provide recommendations to the U.S. Federal Aviation Administration (FAA) on the most significant human factors and the relative contribution of these factors to aviation safety risk.
Reimer, who has worked at MIT since 2003, joins a committee whose operational or academic expertise includes air carrier operations, air traffic control, pilot experience, aeronautical information, aircraft maintenance and mechanics psychology, human-machine integration, and general aviation operations. Their recommendations to the FAA will help ensure safety for passengers, aircraft crews, and cargo for years to come. His appointment follows a year of serving on the Transforming Transportation Advisory Committee (TTAC) for the U.S. Department of Transportation, where he has taken on the role of vice chair on the Artificial Intelligence subcommittee. The TTAC recently released a report to the Secretary of Transportation in response to its charter.
As a mobility and technology futurist working at the intersection of technology, human behavior, and public policy, Reimer brings his expertise in human-machine integration, transportation safety, and AI to the committee. The committee, chartered by congressional mandate through the bipartisan FAA Reauthorization Act of 2024, specifically calls for a portion of the committee to have expertise on human factors but whose experience and training are not primarily in aviation, which Reimer will provide.
MIT CTL creates supply chain innovation and drives it into practice through the three pillars of research, outreach, and education, working with businesses, government, and nongovernmental organizations. As a longtime advocate of collaboration across public and private sectors to ensure consumers’ safety in transportation, Reimer’s particular expertise will help the FAA more broadly consider the human element of aviation safety. Yossi Sheffi, director of MIT CTL, says, “Aviation plays a critical role in the rapid and reliable transportation of goods across vast distances, making it essential for delivering time-sensitive products globally. We must understand the current human factors involved in this process to help ensure smooth operation of this indispensable service amid potential disruptions.”
Reimer recently discussed his research on an episode of The Ojo-Yoshida Report with Phil Koopman, a professor of electrical and computer engineering.
HF Task Force ARC members will serve a two-year term. The first ARC plenary meeting was held Jan. 15-16 in Washington.