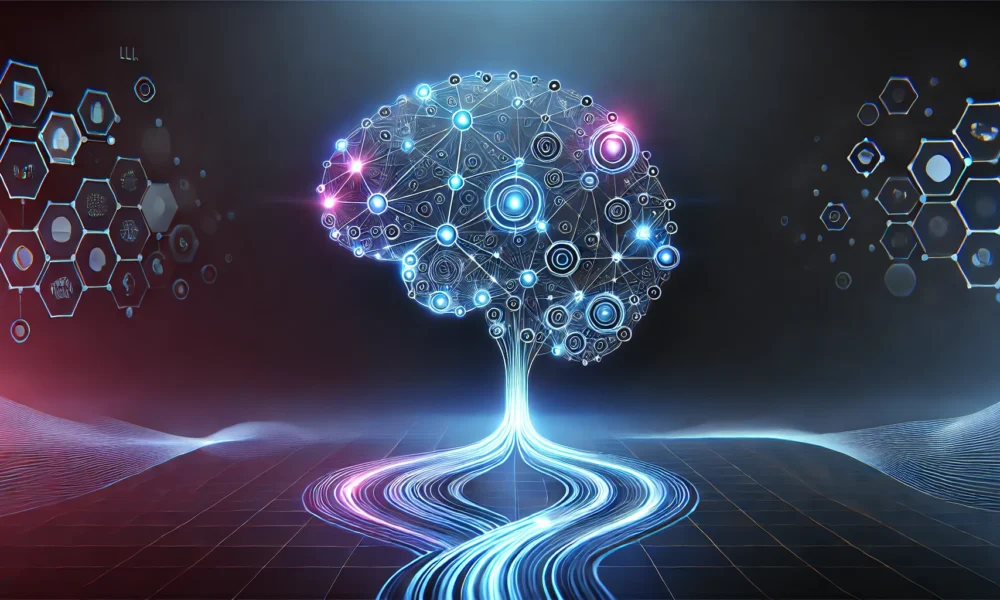
With its cute whale logo, the recent release of DeepSeek could have amounted to nothing more than yet another ChatGPT knockoff. What made it so newsworthy – and what sent competitors’ stocks into a tailspin – was how little it cost to create. It effectively threw a monkey wrench into the U.S.’s notion of the investment it takes to train a high-functioning Large Language Model (LLM).
DeepSeek purportedly spent just $6 million to train its AI model. Juxtapose that with the reported $80–$100 million that OpenAI spent on Chat GPT-4 or the $1 billion they’ve set aside for GPT-5. DeepSeek calls that level of investment into question and leaves big players like Nvidia – whose stock’s value plunged $600 billion in one day – TSMC and Microsoft fretful about AI’s long-term financial viability. If it’s possible to train AI models for significantly less than previously assumed, what does this portend for AI spending overall?
Though the disruption of DeepSeek has led to important discussions, some key points seem to be getting lost in the shuffle. However, what the news brings up is a greater focus on how much innovation costs and the possible economic impact of AI. Here are three important insights arising from this news:
1. DeepSeek’s $6 Million Price Tag is Misleading
Companies need to grasp their infrastructure’s total cost of ownership (TCO). Though DeepSeek’s $6 million price tag has been thrown around a lot, that is probably the cost of just its pre-training run rather than its entire investment. The total cost – not only of running, but of building and training DeepSeek – is likely much higher. Industry analyst firm SemiAnalysis revealed that the company behind DeepSeek spent $1.6 billion on hardware to make its LLM a reality. So, the likely cost is somewhere in the middle.
Whatever the true cost is, the advent of DeepSeek has created a focus on cost-efficient innovation that could be transformational. Innovation is often spurred on by limitations, and the success of DeepSeek underscores the way innovation can happen when engineering teams optimize their resources in the face of real-world constraints.
2. Inference Is What Makes AI Valuable, Not Training
It’s important to pay attention to how much AI model training costs, but training represents a small portion of the overall cost to build and run an AI model. Inference — the manifold ways AI changes how people work, interact, and live — is where AI becomes truly valuable.
This brings up the Jevons paradox, an economic theory suggesting that as technological advancements make the use of a resource more efficient, the overall consumption of that resource may actually increase. In other words, as training costs go down, inference and agentic consumption will increase, and overall spending will follow suit.
AI efficiency may, in fact, lead to a rising tide of AI spending, which should lift all boats, not just Chinese ones. Assuming they ride the efficiency wave, companies like OpenAI and Nvidia will benefit, too.
3. What Remains True is That Unit Economics Matter Most
Making AI more efficient is not merely about lowering costs; it’s also about optimizing unit economics. The Motley Fool forecasts that this year will be the year of AI efficiency. If they’re right, companies should pay attention to lowering their AI training costs as well as their AI consumption costs.
Organizations that build or use AI need to know their unit economics rather than singling out impressive figures like DeepSeek’s $6 million training cost. Real efficiency entails allocating all costs, tracking AI-driven demand, and keeping constant tabs on cost-to-value.
Cloud unit economics (CUE) has to do with measuring and maximizing profit driven by the cloud. CUE compares your cloud costs with revenue and demand metrics, revealing how efficient your cloud spending is, how that has changed over time, and (if you have the right platform) the best ways to increase that efficiency.
Understanding CUE has even greater utility in an AI context, given it is inherently more expensive to consume than traditional cloud services sold by the hyperscalers. Companies building agentic applications could calculate their cost per transaction (e.g. cost per bill, cost per delivery, cost per trade, etc.) and use this to assess the return on investment of specific AI-driven services, products, and features. As AI spending increases, companies will be forced to do this; no company can throw endless dollars at experimental innovation forever. Eventually, it has to make business sense.
Toward Greater Efficiency
However meaningful the $6 million figure is, DeepSeek may have provided a watershed moment that wakes up the tech industry to the inevitable importance of efficiency. Let’s hope this opens the floodgates for cost-effective training, inference, and agentic applications that unlock the true potential and ROI of AI.