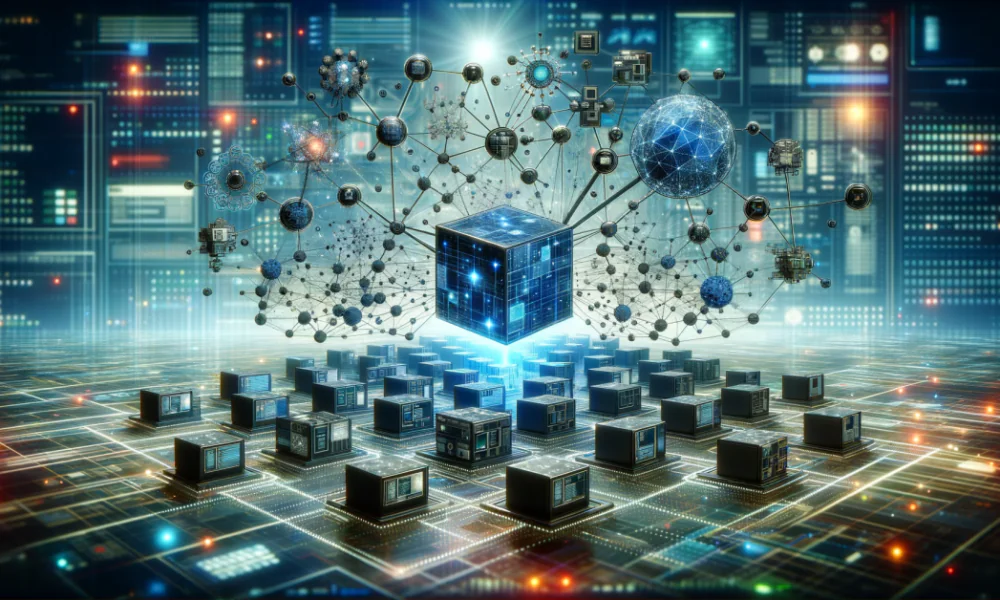
So much of the conversation on AI development has become dominated by a futuristic and philosophical debate – should we approach general artificial intelligence, where AI will become advanced enough to perform any task the way a human could? Is that even possible?
While the acceleration versus deceleration discussion is important and timely with advancements like the Q-star model, other aspects matter, too. Mainly, the importance of decentralizing your technology stack, and how to do that without making it too much of a cost burden. These two challenges can feel at odds: building and deploying models is incredibly expensive, but over-relying on one model can be detrimental in the long run. I know this challenge personally as an AI founder.
To build intelligence, you need talent, data, and scalable compute. To accelerate time to market and do more with less, many companies will choose to build on top of existing models, rather than build from the ground up. And the approach makes sense when what you’re building is so resource-intensive. Compounding this challenge is that, unlike software, most of the gains so far in AI have been made by adding more scale, which requires more computing power and therefore cost.
But what happens when the company in which you’ve built your solution experiences a governance failure or a product outage? From a practical standpoint, relying on a single model to build your product means that you are now part of a negative ripple effect for anything that happens.
We also have to remember the risks of working with systems that are probabilistic. We are not used to this and the world we live in so far has been engineered and designed to function with a definitive answer. Models are fluid in terms of output, and companies constantly tweak the models as well, which means the code you have written to support these and the results your customers are relying on can change without your knowledge or control.
Centralization also creates safety concerns because it introduces a single point of failure. Every company is working in the best interest of itself. If there is a safety or risk concern with a model, you have much less control over fixing that issue or less access to alternatives.
Where does that leave us?
AI is indisputably going to improve how we live. There is so much that it is capable of achieving and fixing, from how we gather information to how we understand vast amounts of data. But with that opportunity also comes risk. If we over-rely on a single model, all companies are opening themselves up to both safety and product challenges.
To fix this, we need to bring the inference costs down and make it easier for companies to have a multi-model approach. And of course, everything comes to data. Data and data ownership will matter. The more unique, high quality, and available the data, the more useful it will be.
For many problems, you can optimize models for a specific application. The last mile of AI is companies building routing logic, evaluations, and orchestration layers on top of these different models, specializing them for different verticals.
There have been multiple substantial investments in this space that are getting us closer to this goal. Mistal’s recent (and impressive) funding round is a promising development towards an OpenAI alternative. There are also companies helping other AI providers make cross-model multiplexing a reality and reducing inference costs via specialized hardware, software, and model distillation, as a few examples.
We are also going to see open-source take off, and government bodies must enable open source to remain open. With open-source models, it’s easier to have more control. However, the performance gaps are still there.
I presume we will end up in a world where you will have junior models optimized to perform less complex tasks at scale while larger super-intelligent models will act as oracles for updates and will increasingly spend compute on solving more complex problems. You will not need a trillion-parameter model to respond to a customer service request. I liken it to not having a senior executive manage a task that an intern can handle. Much like we have multiple roles for human counterparts, most companies will also rely on a collection of models with various levels of sophistication.
To achieve this balance, you need a clear task breakdown and benchmarking, considering the time, computational complexity, cost, and required scale. Depending on the use case, you can prioritize accordingly. Determine a ground truth, an ideal outcome for comparison, and an example input and output data, so you can run various prompts to optimize and get the closest outcome to the ground truth.
If AI companies can successfully decentralize their tech stack and build on multiple models, we can improve the safety and reliability of these tools and thereby maximize the positive impact of AI. We are no longer in a place for theoretical debates – it’s time to focus on how to put AI to work to make these technologies more effective and resilient.