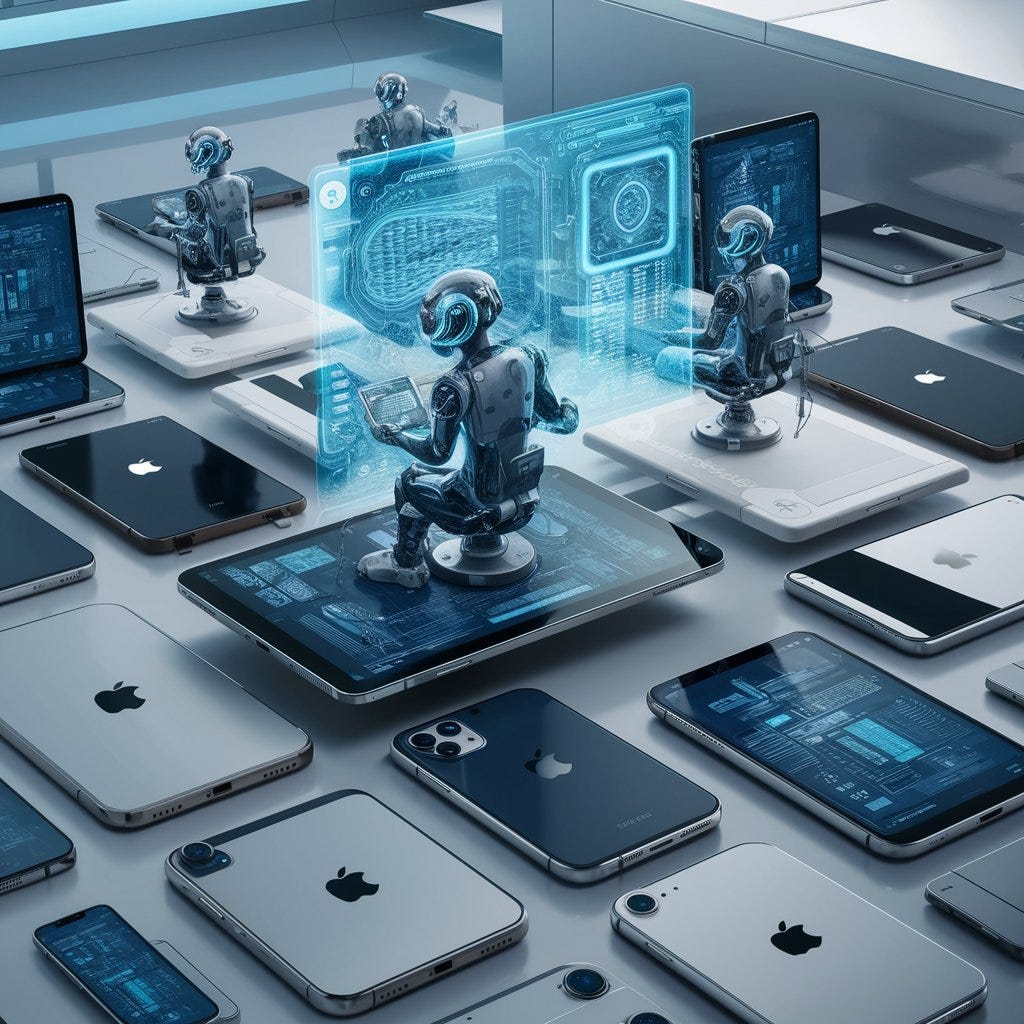
Plus a new super coder model, Meta’s new AI releases, DeepMind’s video-to-audio models and much more.
Next Week in The Sequence:
-
Edge 405: Our series about autonomous agents dives into short term memory augmentation in agents. We discuss Google’s recent Inifini-Attention architecture for virtually unlimited context windows and dives into the famous AutoGPT framework.
-
Edge 406: We dive into OpenAI’s recently published paper on AI interpretability.
You can subscribe to The Sequence below:
📝 Editorial: Beyond OpenAI: Apple’s On-Device AI Strategy
The partnership between Apple and OpenAI dominated the headlines of the recent WWDC conference and sparked passionate debates within the AI community. Some view this partnership as a way to enable best-in-class AI in iOS devices, while others consider it a consequence of Apple’s lack of readiness to build proprietary AI models. The latter assumption would be a mistake. While it is true that Apple hasn’t historically developed the same AI research talent as Microsoft, Google, or Meta, things are rapidly changing, and last week was a validation of that.
A few days ago, Apple open-sourced 20 small models and 4 datasets across different language and image categories. The models are compatible with Apple’s CoreML framework, designed to run on-device models. In the new release, we can find models such as FastViT for image classification, DepthAnything for monocular depth estimation, and DETR for semantic segmentation. These models can run on-device without the need for an Internet connection.
The demand for smaller foundation models that can run on edge devices continues to grow. Several factors are contributing to this. First, mobile and IoT devices represent a significant percentage of user-computer interactions and, as a result, a fertile ground for AI. Computational constraints, personalization requirements, and privacy and security challenges prevent these scenarios from being solved by mega-large foundation models.
Apple has one of the largest distribution channels for on-device models and, consequently, it shouldn’t be a surprise that it is advancing research in that area. Thinking that Apple’s AI strategy is dependent on the partnership with OpenAI would be a mistake. On-device AI is going to be a relevant trend, and Apple one of its main influencers.
🔎 ML Research
DeepMind V2A
Google DeepMind published the research related to their video-to-audio(V2A) models. V2A combines video pixels and prompts to generate rich soundtracks that complement the video clips —> Read more.
DeepSeek-Coder-V2
DeepSeek published the research behind DeepSeek-Coder-v2, a mixture-of-experts(MoE) architecture optimized for coding and math reasoning. DeepSeek-Coder-v2 supports 338 programming languages and shows performance comparable to GPT-4o in coding tasks —> Read more.
Vulnerabilities in Multimodal Agents
Researchers from Carnegie Mellon University(CMU) published a paper outlining a series of adversarial attacks in vision-language agents. The research use adversarial prompts to perturb the model gradients and guide it to take the wrong actions —> Read more.
DataComp
Researchers from several research labs including University of Washington and Apple published a paper unveiling DataComp for Language Models (DCLM), a method for creating high quality training datasets for foundation models. DCLM also introduces a corpus of 240T tokens extracted from Common Crawl and 53 evaluation recipes —> Read more.
Task-Me-Anything
Researchers from the University of Washington and Allen AI published a paper outlining Task-Me-Anything, a technique that is able to generate a benchmark tailored to user’s needs.. The method is optimized for multimodal models and maintains a library of assets across different mediums( videos, images, 3Ds) and combines them to generate new benchmarks —> Read more.
Whiteboard-of-Thought
Researchers from Columbia University published a paper introducing Whiteboard-of-Thought a reasoning method for multimodal models. The core idea is to prompt models with a metaphorical whiteboard that illustrates reasoning steps visually —> Read more.
🤖 Cool AI Tech Releases
Meta New Models
Meta released new models for audio, watermarking, multi-token prediction, images and others —> Read more.
Apple New Models
Apple released a series of small models for language and image capabilities —> Read more.
Claude 3.5 Sonnet
Anthropic released Claude 3.5 Sonnet which exhibit strong performance at much faster speed —> Read more.
Gen-3 Alpha
Runway unveiled Gen-3 Alpha, its new video generation model with tangible fidelity and consistency improvements over its predecessors —> Read more.
AutoGen Studio
Microsoft released AutoGen Studio, a low-code interface for building and testing multi-agent solutions —> Read more.
BigCodeBench
HuggingFace released BigCodeBench, a new benchmark specialized in code-generation tasks —> Read more.
🛠 Real World AI
Model Training at Meta
Meta shares some details about the infrastructure used to scale the training of foundation models internally —> Read more.
Video Classification at Netflix
Netflix discusses their use of vision-language models and active learning to build video classifiers —> Read more.
📡AI Radar
-
OpenAI co-founder Ilya Sutskever, launched a new company called Safe Super intelligence (SSI).
-
Poolside.ai is raising an astonishing $400 million for building a next generation coding copilot.
-
OpenAI acquired Rockset to improve its retrieval capabilities.
-
AI language tutoring app Speak raised $20 million in fresh funding.
-
Day.ai came out of stealth mode with $4 million to build an AI-first CRM.
-
Sierra published a benchmark optimized to evaluate the capabilities of autonomous agents.
-
Customer service agent platform Decagon emerged from stealth mode with $35 million in funding.
-
AI financial data company Finbourne raised $70 million.
-
GrayMatter raised $45 million to streamline robot programming for manufacturing.