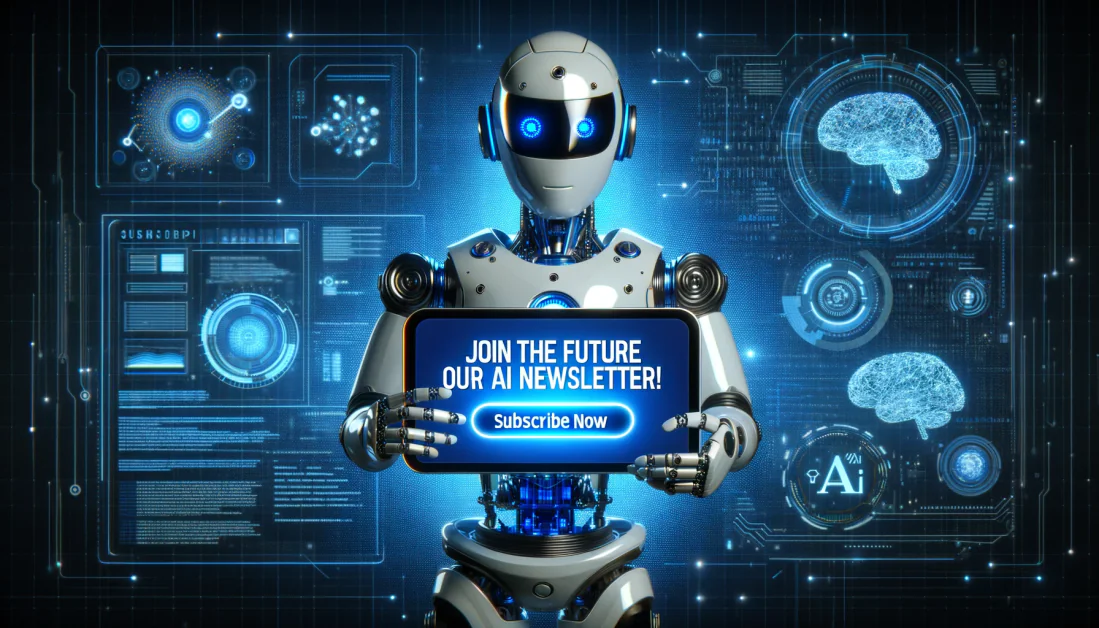
AI, by design, has a “mind of its own.” One drawback of this is that Generative AI models will occasionally fabricate information in a phenomenon called “AI Hallucinations,” one of the earliest examples of which came into the spotlight when a New York judge reprimanded lawyers for using a ChatGPT-penned legal brief that referenced non-existent court cases. More recently, there have been incidents of AI-generated search engines telling users to consume rocks for health benefits, or to use non-toxic glue to help cheese stick to pizza.
As GenAI becomes increasingly ubiquitous, it is important for adopters to recognize that hallucinations are, as of now, an inevitable aspect of GenAI solutions. Built on large language models (LLMs), these solutions are often informed by vast amounts of disparate sources that are likely to contain at least some inaccurate or outdated information – these fabricated answers make up between 3% and 10% of AI chatbot-generated responses to user prompts. In light of AI’s “black box” nature – in which as humans, we have extraordinary difficulty in examining just exactly how AI generates its results, – these hallucinations can be near impossible for developers to trace and understand.
Inevitable or not, AI hallucinations are frustrating at best, dangerous, and unethical at worst.
Across multiple sectors, including healthcare, finance, and public safety, the ramifications of hallucinations include everything from spreading misinformation and compromising sensitive data to even life-threatening mishaps. If hallucinations continue to go unchecked, the well-being of users and societal trust in AI systems will both be compromised.
As such, it is imperative that the stewards of this powerful tech recognize and address the risks of AI hallucinations in order to ensure the credibility of LLM-generated outputs.
RAGs as a Starting Point to Solving Hallucinations
One method that has risen to the fore in mitigating hallucinations is retrieval-augmented generation, or RAG. This solution enhances LLM reliability through the integration of external stores of information – extracting relevant information from a trusted database chosen according to the nature of the query – to ensure more reliable responses to specific queries.
Some industry experts have posited that RAG alone can solve hallucinations. But RAG-integrated databases can still include outdated data, which could generate false or misleading information. In certain cases, the integration of external data through RAGs may even increase the likelihood of hallucinations in large language models: If an AI model relies disproportionately on an outdated database that it perceives as being fully up-to-date, the extent of the hallucinations may become even more severe.
AI Guardrails – Bridging RAG’s Gaps
As you can see, RAGs do hold promise for mitigating AI hallucinations. However, industries and businesses turning to these solutions must also understand their inherent limitations. Indeed, when used in tandem with RAGs, there are complementary methodologies that should be used when addressing LLM hallucinations.
For example, businesses can employ real-time AI guardrails to secure LLM responses and mitigate AI hallucinations. Guardrails act as a net that vets all LLM outputs for fabricated, profane, or off-topic content before it reaches users. This proactive middleware approach ensures the reliability and relevance of retrieval in RAG systems, ultimately boosting trust amongst users, and ensuring safe interactions that align with a company’s brand.
Alternatively, there’s the “prompt engineering” approach, which requires the engineer to change the backend master prompt. By adding pre-determined constraints to acceptable prompts – in other words, monitoring not just where the LLM is getting information but how users are asking it for answers as well – engineered prompts can guide LLMs toward more dependable results. The main downside of this approach is that this type of prompt engineering can be an incredibly time-consuming task for programmers, who are often already stretched for time and resources.
The “fine tuning” approach involves training LLMs on specialized datasets to refine performance and mitigate the risk of hallucinations. This method trains task-specialized LLMs to pull from specific, trusted domains, improving accuracy and reliability in output.
It is also important to consider the impact of input length on the reasoning performance of LLMs – indeed, many users tend to think that the more extensive and parameter-filled their prompt is, the more accurate the outputs will be. However, one recent study revealed that the accuracy of LLM outputs actually decreases as input length increases. Consequently, increasing the number of guidelines assigned to any given prompt does not guarantee consistent reliability in generating dependable generative AI applications.
This phenomenon, known as prompt overloading, highlights the inherent risks of overly complex prompt designs – the more broadly a prompt is phrased, the more doors are opened to inaccurate information and hallucinations as the LLM scrambles to fulfill every parameter.
Prompt engineering requires constant updates and fine-tuning and still struggles to prevent hallucinations or nonsensical responses effectively. Guardrails, on the other hand, won’t create additional risk of fabricated outputs, making them an attractive option for protecting AI. Unlike prompt engineering, guardrails offer an all-encompassing real-time solution that ensures generative AI will only create outputs from within predefined boundaries.
While not a solution on its own, user feedback can also help mitigate hallucinations with actions like upvotes and downvotes helping refine models, enhance output accuracy, and lower the risk of hallucinations.
On their own, RAG solutions require extensive experimentation to achieve accurate results. But when paired with fine-tuning, prompt engineering, and guardrails, they can offer more targeted and efficient solutions for addressing hallucinations. Exploring these complimentary strategies will continue to improve hallucination mitigation in LLMs, aiding in the development of more reliable and trustworthy models across various applications.
RAGs are Not the Solution to AI Hallucinations
RAG solutions add immense value to LLMs by enriching them with external knowledge. But with so much still unknown about generative AI, hallucinations remain an inherent challenge. The key to combating them lies not in trying to eliminate them, but rather by alleviating their influence with a combination of strategic guardrails, vetting processes, and finetuned prompts.
The more we can trust what GenAI tells us, the more effectively and efficiently we’ll be able to leverage its powerful potential.