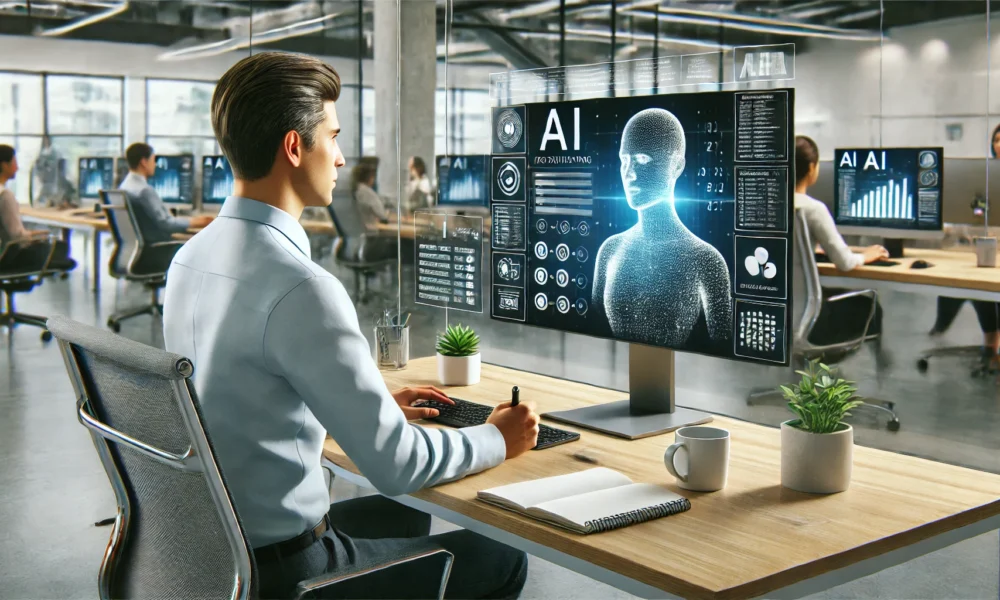
AI tools have seen widespread business adoption since ChatGPT’s 2022 launch, with 98% of small businesses surveyed by the US Chamber of Commerce using them. However, despite success in areas like data analysis, summarization, personalization and others, a recent survey of 2,500 workers across the US, UK, Australia, and Canada found that 3 out of 4 workers report AI has actually increased their workloads. The promise of AI therefore remains high, but the reality on the ground seems so far to be slightly underwhelming.
This discrepancy underscores a critical challenge: bridging the gap between AI’s vast promise and its currently limited practical impact on enterprise operations. Closing this gap is essential for organizations to fully realize the value of their AI investments and grow adoption among their workers and stakeholders.
A product vision for AI investments
While AI has made significant strides, many business solutions remain at the experimental proof-of-concept stage and are not fully suited for day-to-day operations. In a cross-country and industry survey of 1,000 CxOs and senior executives, BCG found that 74% of companies struggle to realize and scale value in their AI investments. Part of the reason for this is that today, the most prominent AI user interfaces are based on natural language delivered through a chatbot paradigm. While these modalities are undoubtedly useful when it comes to tasks like summarization and other text-based contexts, they fail to match up with how work is actually conducted in most enterprises.
To maximize impact, the design of AI tools must evolve to go beyond isolated, text-based interfaces into integrated, workflow-enhancing applications that better meet the operational needs of large organizations. The next phase of AI evolution will increasingly be agentic, blending seamlessly into the background of enterprise operations and allowing teams to focus on high-level ideation and strategy leading into automated operations, bypassing manual execution but still retaining the human-in-the-loop control that still relies on non-automatable human judgment.
This transition from “experimental” to “essential” requires a productized approach to AI development, deployment, and operations, akin to how Apple for example revolutionized the tech industry with the launch of the iPhone—a thoughtfully designed, user-friendly product that integrated state-of-the-art technology and married it to a world-class user experience from day one.
Closing data gaps and ensuring cost efficiencies
In order to move towards this more sophisticated productized version of AI, it’s vital to tackle the gaps within the enterprise data estate. The increasing interest in deploying AI in enterprises has exposed widespread data silos, which hinder organizations from scaling AI beyond prototypes.
Of course, it’s important to note that financial hurdles can also deter organizations from expanding their AI use from pilots to enterprise-wide applications. The infrastructure required for training and maintaining advanced AI models—spanning computing power, data storage, and ongoing operational costs—can escalate quickly. Without careful oversight, these projects risk becoming unsustainably expensive, mirroring the early challenges seen during the adoption of cloud technologies.
Focusing on ensuring the integrity, cleanliness, and quality of data in the first instance can help keep costs down in the long run. Too often, companies focus on AI first and address their data challenges only later, creating inefficiencies and missed opportunities.
Cost efficiency is closely tied to investments across the data and core infrastructure layer. Investing in this portion of the stack is key to ensuring LLMs can be run at scale. In practical terms, this means standardizing data collection, ensuring accessibility, and implementing robust data governance frameworks.
Responsible AI
Companies that embed responsible AI principles on a robust, well-governed data foundation will be better positioned to scale their applications efficiently and ethically. Principles such as fairness, transparency, and accountability in AI inputs and outputs are no longer optional for enterprises—they are strategic imperatives for keeping trust with employees and customers, as well as complying with emerging regulations.
One critical framework is the EU AI Act, which mandates clear documentation, transparency, and governance for high-risk AI systems. Compliance with such frameworks requires companies to implement processes that not only validate their AI models but also make them interpretable and accountable, which is particularly vital in high-stakes applications like credit scoring, fraud detection, and investment recommendations. Firms that prioritize these practices can stay ahead of regulatory demands and avoid costly legal or reputational risks.
Moreover, as the industry evolves and agentic AI systems that can make autonomous decisions become more widespread, the stakes for responsible implementation grow higher. Delegating actions to AI tools requires confidence in their reliability and ethical behavior. To achieve this, organizations must invest in continuous auditing and monitoring frameworks to ensure that AI systems operate as intended, and guard judiciously against outcome biases and perpetuating unfair outcomes.
Looking ahead
The transformative potential of AI in enterprise operations is undeniable, but realizing its full value requires a shift in how organizations approach its development and deployment. Moving beyond experimental applications to scalable, workflow-integrated tools necessitates a keen focus on addressing foundational issues of data quality, governance, and accessibility, and adopting a product mindset.
Closing data gaps and making Responsible AI a centerpiece of strategy will be key to maintaining trust with stakeholders, continuing to meet strategic compliance imperatives, and ensuring AI systems are not only scalable but also reliable and effective. In this way, the promise of AI can be realized and its current adoption struggles will be overcome at organizations of every size.