Although AutoML rose to popularity a few years ago, the ealy work on AutoML dates back to the early 90’s when scientists published the first papers on hyperparameter optimization. It was in 2014 when ICML organized the first AutoML workshop that AutoML gained the attention of…
Hallucination Control: Benefits and Risks of Deploying LLMs as Part of Security Processes
Large Language Models (LLMs) trained on vast quantities of data can make security operations teams smarter. LLMs provide in-line suggestions and guidance on response, audits, posture management, and more. Most security teams are experimenting with or using LLMs to reduce manual toil in workflows. This can…
Jonathan Corbin, Founder & CEO of Maven AGI – Interview Series
Jonathan Corbin, is the Founder & CEO of Maven AGI. Previously, as the Global Vice President of Customer Success & Strategy at HubSpot, Jonathan led a team of approximately 1,000 customer success, partner success, and contract managers across multiple regions and verticals. His responsibilities included driving…
Qwen2 – Alibaba’s Latest Multilingual Language Model Challenges SOTA like Llama 3
After months of anticipation, Alibaba’s Qwen team has finally unveiled Qwen2 – the next evolution of their powerful language model series. Qwen2 represents a significant leap forward, boasting cutting-edge advancements that could potentially position it as the best alternative to Meta’s celebrated Llama 3 model. In…
5 ways generative AI will impact CISOs & cyber security teams – CyberTalk
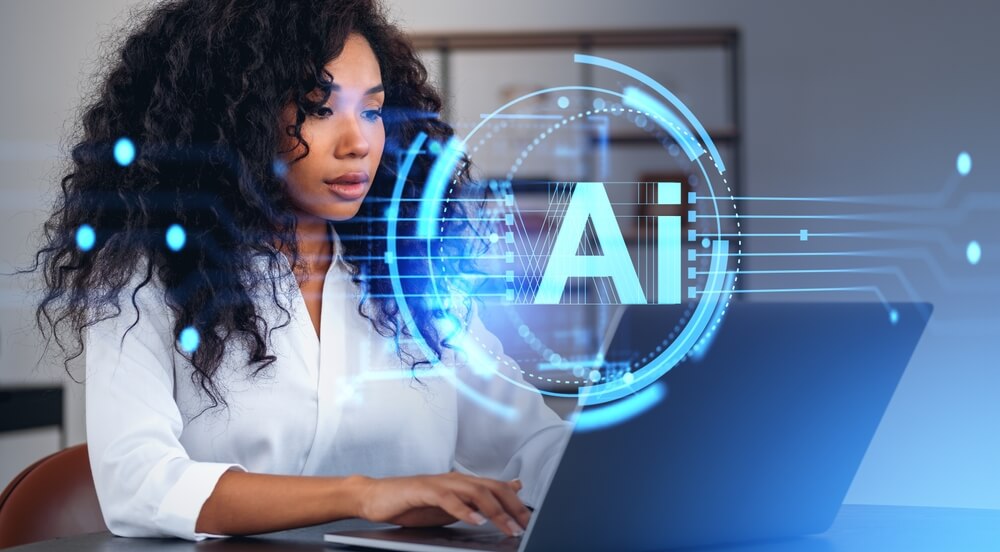
EXECUTIVE SUMMARY:
Enterprises and individuals have adopted generative AI at an extremely impressive rate. In 2024, generative AI is projected to reach 77.8 million users worldwide — an adoption rate of more than double that of smartphones and tablets across a comparable time frame.
While the integration of generative AI into work environments offers coveted agility and productivity gains, such benefits remain tenuous without the right workforce (and societal) structures in-place to support AI-driven growth.
It nearly goes without saying — Generative AI introduces a new layer of complexity into organizational systems. Effective corresponding workplace transformation — one that enables people to use generative AI for efficiency and productivity gains — depends on our abilities to secure it, secure our people, and secure our processes.
In the second half of 2024, CISOs and cyber security teams can facilitate the best possible generative AI-based business outcomes by framing discussions and focal points around the following:
5 ways generative AI will impact CISOs and security teams
1. Expanded responsibilities. It should have been written on a neon sign…Generative AI will add new ‘to-dos’ to CISOs’ (already extensive) list of responsibilities. Only 9% of CISOs say that they are currently prepared to manage the risks associated with generative AI.
New generative AI-related responsibilities will involve dealing with data security and privacy, access control, model integrity and security, and user training, among other things.
2. AI governance. As generative AI’s footprint expands within enterprises, cyber security leaders must develop comprehensive governance frameworks to mitigate corresponding risks.
This includes addressing the potential for “shadow generative AI,” referring to the unsanctioned use of generative AI tooling. Shadow generative AI poses challenges that parallel those associated with shadow IT.
To build a strategic AI governance plan for your organization, start with an assessment of your organization’s unique needs and generative AI use-cases.
3. User training. Successful AI governance hinges on effective user awareness and training initiatives. Currently, only 17% of organizations have fully trained their teams on the risks around generative AI.
Prioritize generative AI awareness programs, as to communicate acceptable and unacceptable use-cases. This ultimately minimizes the potential for painful cyber security stumbles.
4. The dual-use dilemma. This concept refers to the notion that generative AI technologies can be applied for both beneficial and malicious gain.
The overwhelming majority of CISOs (70%) believe that generative AI will lead to an imbalance in “firepower,” enabling the cyber criminals to wreak havoc on organizations at an unprecedented rate.
Will AI-generated phishing emails achieve a higher click-through rates and perpetuate a high volume of attacks? No one knows. In the interim, CISOs are advised to proactively update and upgrade cyber security technologies.
5. AI in security tooling. Just over a third of CISOs currently use AI — either extensively, or peripherally — within cyber security functions. However, within the next 12 months, 61% of CISOs intend to explore opportunities for generative AI implementation in security processes and protocols.
If your organization is currently assessing AI-based cyber security threat prevention technologies, see how Check Point’s Infinity AI Copilot can advance your initiatives. Learn more here.
Also, be sure to check out this CISO’s Guide to AI. Lastly, to receive cyber security thought leadership articles, groundbreaking research and emerging threat analyses each week, subscribe to the CyberTalk.org newsletter.
The 10 Best Dedicated Hosting Providers in 2024
Dedicated hosting is the highest tier of web hosting – you get a whole server to yourself and don’t need to share the server’s resources with any other website owner. The perks? Exceptional website performance, unlimited bandwidth, exclusive IP address, infinite flexibility, and unmatched security. However,…
Digital Humans Are Not Just AI with a Face
Digital humans used to be simple chatbots that often misunderstood questions, which many people found frustrating. Now, they’ve evolved into advanced virtual agents that can communicate as effectively as the best customer service representatives, possess expert-level knowledge, and look strikingly like real humans. These advanced digital…
Josh Wong, Founder & CEO of ThinkLabs AI – Interview Series
Josh Wong is the Founder and CEO of ThinkLabs AI. He previously worked at GE Vernova as a General Manager, Grid Orchestration. Josh Wong attended the University of Waterloo. ThinkLabs AI is a specialized AI development and deployment company. Its mission is to empower critical industries…
Med-Gemini: Transforming Medical AI with Next-Gen Multimodal Models
Artificial intelligence (AI) has been making waves in the medical field over the past few years. It’s improving the accuracy of medical image diagnostics, helping create personalized treatments through genomic data analysis, and speeding up drug discovery by examining biological data. Yet, despite these impressive advancements,…
Apple WWDC: Unleashing the Power of AI and Spatial Computing with Groundbreaking Updates
The recent Apple Worldwide Developers Conference (WWDC) showcased significant updates across Apple’s platforms, introducing new features and enhancements designed to elevate user experience and developer capabilities. The event highlighted advancements in AI, updates to various operating systems, and notable improvements in Apple’s hardware and services. Key…