Infinity Nikki’s big PlayStation State of Play spotlight in May garnered an array of reactions. Fans of the popular Nikki series of mobile fashion games were excited to see the pink-haired fashionista make her triple-A debut. Unfamiliar viewers either dismissed it as an overly whimsical dress-up game or found themselves unexpectedly intrigued by its delightful and unique spin on an open-world experience. I count myself in that third camp. Something about the game’s premise stuck with me, but after playing several hours of a beta version, I’ve gone from admiring Infinity Nikki conceptually to being genuinely excited to wear the full experience.
The adventure sees the titular Nikki and her furry best pal, Momo, preparing for a ball only to be unwillingly transported to the magical world of Miraland. A god-like being named Ena the Curator tells Nikki she’s been chosen to find and restore the Miracle Outfits, a collection of magical and powerful dresses, to uncover a divine truth. Along the way, Nikki also learns that she’s a Stylist. These are special people with the ability to find and create outfits anytime, anywhere.
I begin the game with three Ability Dresses, special outfits bestowing a unique power. One is the blue dress/blonde hair ensemble seen in the State of Play trailer that allows me to perform a floating jump Princess Peach style. Not only is this good for crossing large gaps or bounding between rooftops, but Nikki can also perform a plummeting slam to defeat enemies or shatter fragile objects. A Purification dress is used for combat, letting Nikki fire orbs of cleansing energy to purify (not kill) demonically corrupted enemies called Esselings and collectibles. One cute dress lets Nikki groom certain animals to collect materials from them. Primary abilities like floating and purification are mapped to buttons while specialized dresses like the grooming or a bug-catching dress I unlock later can be activated via a selection wheel.
[embedded content]
Based on the beta slice, Miraland is vast and inviting, thanks to colorful and lush flower fields, rolling hills, and the quaint village of Florawish. Infinity Nikki looks as pretty as her wardrobe. There’s plenty to do and explore, whether it’s completing side quests or, the main hook, hunting for Whimstars. These special stars are used to unlock new items and perks from a skill tree and are scattered everywhere. While some can merely be found (with help from Momo’s special vision that can highlight and tag distant Whimstars), others have small challenges tied to them. Examples include defeating enemies or searching for hidden gold stars, such as an ornament atop an umbrella. You can also gain Whimstars by entering special portals that warp players to platforming/puzzle challenge rooms reminiscent of shrines from The Legend of Zelda: Breath of the Wild/Tears of the Kingdom, albeit simpler. I like gathering Whimstars as it captures the familiar joy of collecting stars in a 3D Mario game.
Whimstars are spent unlocking new outfits and other items in a skill tree called the Heart of Infinity. However, this only makes the outfit available to be crafted – you still have to make it yourself. While exploring Miraland, you’ll gather materials like various fruits, flowers, threads, furs, and more to craft a desired ensemble, consisting of parts like hairstyles, upper and lower body wear, make-up, and accessories. Sketches for outfits can be found or earned by completing quests and other activities.
The more outfit sketches you find and unlock, the higher your level as a Stylist rises. Early on, Nikki joins the Stylist Guild in Florawish. Here, she receives a tablet-like device called a Pear-Pal that keeps track of a litany of goals, such as defeating a quota of enemies or taking photos with an in-game camera. Completing goals raises your rank, which rewards money and materials to unlock more outfits. This should appeal to objective-oriented players, as there’s no shortage of meters to fill. With main story and side quests, Stylist Rank, and daily challenges, Infinity Nikki constantly tracks and rewards all aspects of play in a manner similar to games like Genshin Impact.
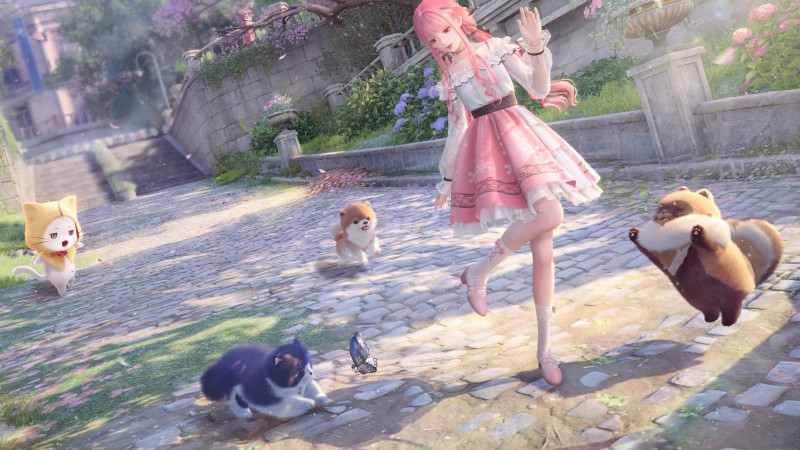
Checking a box usually means getting new crafting materials or outfit sketches. This is an effective hook, as the Nikki series’ Bread and Butter is dressing up the heroine with a staggering collection of clothing options. You can outfit Nikki however you wish, regardless of any abilities tied to outfit pieces. If you really dig the bug-catching dress and want Nikki to rock that look 24/7, you can do that. With so many apparel options, players will likely spend a lot of time making Nikki look as girly, regal, or edgy as they see fit.
Outside of roaming the scenic open world and collecting stars and materials, a main story quest adds some meaty narrative intrigue. Cutscenes are nicely rendered and have solid comedic writing at times. Characters like a talking dragon-like poet or moments like watching a girl riding a giant origami paper plane crash into and level a building add an oddball charm to the perpetually saccharine vibe.
Infinity Nikki is chock full of charm at every turn. Even manipulating the world’s day/night cycle involves playing a cute Flappy Bird-esque minigame. Every corner aims to make you smile while completing myriad objectives, and earning new outfits provide effective dopamine hits. While I enjoy basking in Infinity Nikki’s cozy atmosphere, its cinematic teases of a grander mystery intrigued me even more. I still have little idea what to make of this world and how it works, but I want to learn more, and several lore books and other notes suggest what could be unexpectedly deep world-building. The game also features a multiplayer component, but it was not available in the beta, and developer Infold Games isn’t ready to discuss it yet.
Most of all, having an open-world game that doesn’t feature overt violence is refreshing. I wouldn’t consider any obstacle I’ve encountered thus far to be challenging, but there’s an allure to just being in this world that’s hard to deny – everything is just so darn pleasant. I’ve got my outfit picked out, so hopefully, Infinity Nikki’s release won’t leave us waiting too much longer.
Infinity Nikki is coming to PlayStation 5, PC, iOS, and Android. A closed PC public beta is now available for select registered players.