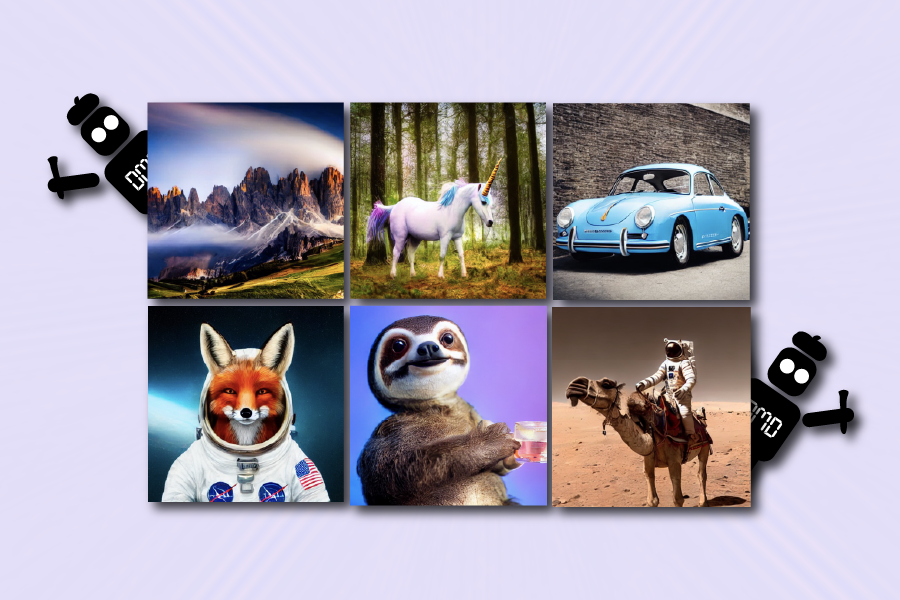
In our current age of artificial intelligence, computers can generate their own “art” by way of diffusion models, iteratively adding structure to a noisy initial state until a clear image or video emerges. Diffusion models have suddenly grabbed a seat at everyone’s table: Enter a few words and experience instantaneous, dopamine-spiking dreamscapes at the intersection of reality and fantasy. Behind the scenes, it involves a complex, time-intensive process requiring numerous iterations for the algorithm to perfect the image.
MIT Computer Science and Artificial Intelligence Laboratory (CSAIL) researchers have introduced a new framework that simplifies the multi-step process of traditional diffusion models into a single step, addressing previous limitations. This is done through a type of teacher-student model: teaching a new computer model to mimic the behavior of more complicated, original models that generate images. The approach, known as distribution matching distillation (DMD), retains the quality of the generated images and allows for much faster generation.
“Our work is a novel method that accelerates current diffusion models such as Stable Diffusion and DALLE-3 by 30 times,” says Tianwei Yin, an MIT PhD student in electrical engineering and computer science, CSAIL affiliate, and the lead researcher on the DMD framework. “This advancement not only significantly reduces computational time but also retains, if not surpasses, the quality of the generated visual content. Theoretically, the approach marries the principles of generative adversarial networks (GANs) with those of diffusion models, achieving visual content generation in a single step — a stark contrast to the hundred steps of iterative refinement required by current diffusion models. It could potentially be a new generative modeling method that excels in speed and quality.”
This single-step diffusion model could enhance design tools, enabling quicker content creation and potentially supporting advancements in drug discovery and 3D modeling, where promptness and efficacy are key.
Distribution dreams
DMD cleverly has two components. First, it uses a regression loss, which anchors the mapping to ensure a coarse organization of the space of images to make training more stable. Next, it uses a distribution matching loss, which ensures that the probability to generate a given image with the student model corresponds to its real-world occurrence frequency. To do this, it leverages two diffusion models that act as guides, helping the system understand the difference between real and generated images and making training the speedy one-step generator possible.
The system achieves faster generation by training a new network to minimize the distribution divergence between its generated images and those from the training dataset used by traditional diffusion models. “Our key insight is to approximate gradients that guide the improvement of the new model using two diffusion models,” says Yin. “In this way, we distill the knowledge of the original, more complex model into the simpler, faster one, while bypassing the notorious instability and mode collapse issues in GANs.”
Yin and colleagues used pre-trained networks for the new student model, simplifying the process. By copying and fine-tuning parameters from the original models, the team achieved fast training convergence of the new model, which is capable of producing high-quality images with the same architectural foundation. “This enables combining with other system optimizations based on the original architecture to further accelerate the creation process,” adds Yin.
When put to the test against the usual methods, using a wide range of benchmarks, DMD showed consistent performance. On the popular benchmark of generating images based on specific classes on ImageNet, DMD is the first one-step diffusion technique that churns out pictures pretty much on par with those from the original, more complex models, rocking a super-close Fréchet inception distance (FID) score of just 0.3, which is impressive, since FID is all about judging the quality and diversity of generated images. Furthermore, DMD excels in industrial-scale text-to-image generation and achieves state-of-the-art one-step generation performance. There’s still a slight quality gap when tackling trickier text-to-image applications, suggesting there’s a bit of room for improvement down the line.
Additionally, the performance of the DMD-generated images is intrinsically linked to the capabilities of the teacher model used during the distillation process. In the current form, which uses Stable Diffusion v1.5 as the teacher model, the student inherits limitations such as rendering detailed depictions of text and small faces, suggesting that DMD-generated images could be further enhanced by more advanced teacher models.
“Decreasing the number of iterations has been the Holy Grail in diffusion models since their inception,” says Fredo Durand, MIT professor of electrical engineering and computer science, CSAIL principal investigator, and a lead author on the paper. “We are very excited to finally enable single-step image generation, which will dramatically reduce compute costs and accelerate the process.”
“Finally, a paper that successfully combines the versatility and high visual quality of diffusion models with the real-time performance of GANs,” says Alexei Efros, a professor of electrical engineering and computer science at the University of California at Berkeley who was not involved in this study. “I expect this work to open up fantastic possibilities for high-quality real-time visual editing.”
Yin and Durand’s fellow authors are MIT electrical engineering and computer science professor and CSAIL principal investigator William T. Freeman, as well as Adobe research scientists Michaël Gharbi SM ’15, PhD ’18; Richard Zhang; Eli Shechtman; and Taesung Park. Their work was supported, in part, by U.S. National Science Foundation grants (including one for the Institute for Artificial Intelligence and Fundamental Interactions), the Singapore Defense Science and Technology Agency, and by funding from Gwangju Institute of Science and Technology and Amazon. Their work will be presented at the Conference on Computer Vision and Pattern Recognition in June.