Nabil Hannan is the Field CISO (Chief Information Security Officer) at NetSPI. He leads the company’s advisory consulting practice, focusing on helping clients solve their cyber security assessment and threat andvulnerability management needs. His background is in building and improving effective software security initiatives, with deep…
Cybord Secures $8.7M in Series A Funding to Revolutionize Electronics Manufacturing with Traceability
Cybord, a company at the forefront of visual AI technology for electronic manufacturing, has raised $8.7 million in a Series A funding round. The funding, led by Capri Ventures with participation from Ocean Azul Partners, IL Ventures, and NextLeap Ventures, will enable Cybord to expand its…
Non-fiction books that explore AI’s impact on society – AI News
Artificial Intelligence (AI) is code or technologies that perform complex calculations, an area that encompasses simulations, data processing and analytics. AI has increasingly grown in importance, becoming a game changer in many industries, including healthcare, education and finance. The use of AI has been proven to…
Epiphan Pearl Mini Approved by HETMA: Exceeds Expectations! – Videoguys
The Pearl family of lecture capture systems is proudly certified by the Higher Education Technology Managers Alliance (HETMA), a testament to their reliability and perfect fit for higher education.
This certification confirms that Pearl devices meet the high standards set by education professionals for lecture capture and streaming.
Faculty friendly, IT approved
Epiphan makes video capture and streaming content simple, so faculty can focus on teaching.

Experience no-touch capture With our no-touch video capture, faculty can focus on their students – not technology. Epiphan also works seamlessly with your schedule, recording locally and pushing content to the cloud. |
Elevate the student experience Give remote students the same, superior experience they would expect in the classroom with high-definition, multi-camera video and high-fidelity audio. |
Make your investments smarter Get real time visibility and control over every video and audio signal on campus, all from the cloud, plus seamless integration with Q-SYS, Crestron, or Extron systems |

Your faculty and IT team are busy
Make their jobs easier with a smart lecture capture system that everyone can agree on
![]() Build a lab Start with a pilot to evaluate how Epiphan solutions works with every investment you’ve already made. |
![]() Measure success |
![]() Scale across campus |
Learn more about Epiphan Pearl Approved by HETMA HERE
Learn more about Epiphan below:
A wobble from Mars could be sign of dark matter, MIT study finds

In a new study, MIT physicists propose that if most of the dark matter in the universe is made up of microscopic primordial black holes — an idea first proposed in the 1970s — then these gravitational dwarfs should zoom through our solar system at least once per decade. A flyby like this, the researchers predict, would introduce a wobble into Mars’ orbit, to a degree that today’s technology could actually detect.
Such a detection could lend support to the idea that primordial black holes are a primary source of dark matter throughout the universe.
“Given decades of precision telemetry, scientists know the distance between Earth and Mars to an accuracy of about 10 centimeters,” says study author David Kaiser, professor of physics and the Germeshausen Professor of the History of Science at MIT. “We’re taking advantage of this highly instrumented region of space to try and look for a small effect. If we see it, that would count as a real reason to keep pursuing this delightful idea that all of dark matter consists of black holes that were spawned in less than a second after the Big Bang and have been streaming around the universe for 14 billion years.”
Kaiser and his colleagues report their findings today in the journal Physical Review D. The study’s co-authors are lead author Tung Tran ’24, who is now a graduate student at Stanford University; Sarah Geller ’12, SM ’17, PhD ’23, who is now a postdoc at the University of California at Santa Cruz; and MIT Pappalardo Fellow Benjamin Lehmann.
Beyond particles
Less than 20 percent of all physical matter is made from visible stuff, from stars and planets, to the kitchen sink. The rest is composed of dark matter, a hypothetical form of matter that is invisible across the entire electromagnetic spectrum yet is thought to pervade the universe and exert a gravitational force large enough to affect the motion of stars and galaxies.
Physicists have erected detectors on Earth to try and spot dark matter and pin down its properties. For the most part, these experiments assume that dark matter exists as a form of exotic particle that might scatter and decay into observable particles as it passes through a given experiment. But so far, such particle-based searches have come up empty.
In recent years, another possibility, first introduced in the 1970s, has regained traction: Rather than taking on a particle form, dark matter could exist as microscopic, primordial black holes that formed in the first moments following the Big Bang. Unlike the astrophysical black holes that form from the collapse of old stars, primordial black holes would have formed from the collapse of dense pockets of gas in the very early universe and would have scattered across the cosmos as the universe expanded and cooled.
These primordial black holes would have collapsed an enormous amount of mass into a tiny space. The majority of these primordial black holes could be as small as a single atom and as heavy as the largest asteroids. It would be conceivable, then, that such tiny giants could exert a gravitational force that could explain at least a portion of dark matter. For the MIT team, this possibility raised an initially frivolous question.
“I think someone asked me what would happen if a primordial black hole passed through a human body,” recalls Tung, who did a quick pencil-and-paper calculation to find that if such a black hole zinged within 1 meter of a person, the force of the black hole would push the person 6 meters, or about 20 feet away in a single second. Tung also found that the odds were astronomically unlikely that a primordial black hole would pass anywhere near a person on Earth.
Their interest piqued, the researchers took Tung’s calculations a step further, to estimate how a black hole flyby might affect much larger bodies such as the Earth and the moon.
“We extrapolated to see what would happen if a black hole flew by Earth and caused the moon to wobble by a little bit,” Tung says. “The numbers we got were not very clear. There are many other dynamics in the solar system that could act as some sort of friction to cause the wobble to dampen out.”
Close encounters
To get a clearer picture, the team generated a relatively simple simulation of the solar system that incorporates the orbits and gravitational interactions between all the planets, and some of the largest moons.
“State-of-the-art simulations of the solar system include more than a million objects, each of which has a tiny residual effect,” Lehmann notes. “But even modeling two dozen objects in a careful simulation, we could see there was a real effect that we could dig into.”
The team worked out the rate at which a primordial black hole should pass through the solar system, based on the amount of dark matter that is estimated to reside in a given region of space and the mass of a passing black hole, which in this case, they assumed to be as massive as the largest asteroids in the solar system, consistent with other astrophysical constraints.
“Primordial black holes do not live in the solar system. Rather, they’re streaming through the universe, doing their own thing,” says co-author Sarah Geller. “And the probability is, they’re going through the inner solar system at some angle once every 10 years or so.”
Given this rate, the researchers simulated various asteroid-mass black holes flying through the solar system, from various angles, and at velocities of about 150 miles per second. (The directions and speeds come from other studies of the distribution of dark matter throughout our galaxy.) They zeroed in on those flybys that appeared to be “close encounters,” or instances that caused some sort of effect in surrounding objects. They quickly found that any effect in the Earth or the moon was too uncertain to pin to a particular black hole. But Mars seemed to offer a clearer picture.
The researchers found that if a primordial black hole were to pass within a few hundred million miles of Mars, the encounter would set off a “wobble,” or a slight deviation in Mars’ orbit. Within a few years of such an encounter, Mars’ orbit should shift by about a meter — an incredibly small wobble, given the planet is more than 140 million miles from Earth. And yet, this wobble could be detected by the various high-precision instruments that are monitoring Mars today.
If such a wobble were detected in the next couple of decades, the researchers acknowledge there would still be much work needed to confirm that the push came from a passing black hole rather than a run-of-the-mill asteroid.
“We need as much clarity as we can of the expected backgrounds, such as the typical speeds and distributions of boring space rocks, versus these primordial black holes,” Kaiser notes. “Luckily for us, astronomers have been tracking ordinary space rocks for decades as they have flown through our solar system, so we could calculate typical properties of their trajectories and begin to compare them with the very different types of paths and speeds that primordial black holes should follow.”
To help with this, the researchers are exploring the possibility of a new collaboration with a group that has extensive expertise simulating many more objects in the solar system.
“We are now working to simulate a huge number of objects, from planets to moons and rocks, and how they’re all moving over long time scales,” Geller says. “We want to inject close encounter scenarios, and look at their effects with higher precision.”
“It’s a very neat test they’ve proposed, and it could tell us if the closest black hole is closer than we realize,” says Matt Caplan, associate professor of physics at Illinois State University, who was not involved in the study. “I should emphasize there’s a little bit of luck involved too. Whether or not a search finds a loud and clear signal depends on the exact path a wandering black hole takes through the solar system. Now that they’ve checked this idea with simulations, they have to do the hard part — checking the real data.”
This work was supported in part by the U.S. Department of Energy and the U.S. National Science Foundation, which includes an NSF Mathematical and Physical Sciences postdoctoral fellowship.
10 Best Data Integration Tools (September 2024)
Data is the core component of effective organizational decision-making. Today, companies generate more data – over 145 zettabytes in 2024 – through sources like social media, Internet-of-Things (IoT) sources, and point-of-sale (POS) systems. The challenge? Compiling data from these disparate systems into one unified location. This…
Multiple Anchors
Only Chris, right? You’ll want to view this in a Chromium browser:
CodePen Embed Fallback
This is exactly the sort of thing I love, not for its practicality (cuz it ain’t), but for how it illustrates a concept. Generally, tutorials …
Multiple Anchors originally published on CSS-Tricks, which is…
Enhancing LLM collaboration for smarter, more efficient solutions
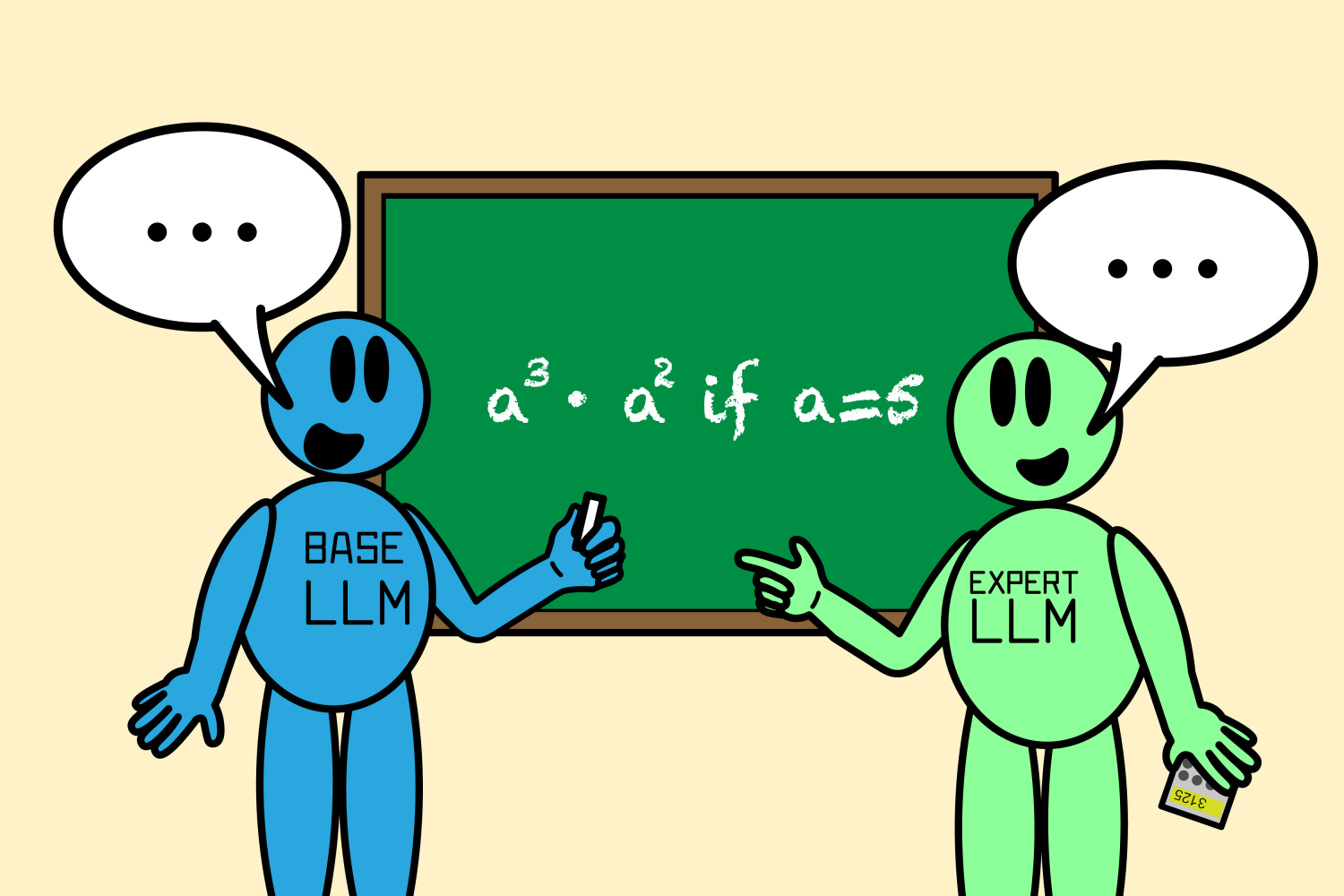
Ever been asked a question you only knew part of the answer to? To give a more informed response, your best move would be to phone a friend with more knowledge on the subject.
This collaborative process can also help large language models (LLMs) improve their accuracy. Still, it’s been difficult to teach LLMs to recognize when they should collaborate with another model on an answer. Instead of using complex formulas or large amounts of labeled data to spell out where models should work together, researchers at MIT’s Computer Science and Artificial Intelligence Laboratory (CSAIL) have envisioned a more organic approach.
Their new algorithm, called “Co-LLM,” can pair a general-purpose base LLM with a more specialized model and help them work together. As the former crafts an answer, Co-LLM reviews each word (or token) within its response to see where it can call upon a more accurate answer from the expert model. This process leads to more accurate replies to things like medical prompts and math and reasoning problems. Since the expert model is not needed at each iteration, this also leads to more efficient response generation.
To decide when a base model needs help from an expert model, the framework uses machine learning to train a “switch variable,” or a tool that can indicate the competence of each word within the two LLMs’ responses. The switch is like a project manager, finding areas where it should call in a specialist. If you asked Co-LLM to name some examples of extinct bear species, for instance, two models would draft answers together. The general-purpose LLM begins to put together a reply, with the switch variable intervening at the parts where it can slot in a better token from the expert model, such as adding the year when the bear species became extinct.
“With Co-LLM, we’re essentially training a general-purpose LLM to ‘phone’ an expert model when needed,” says Shannon Shen, an MIT PhD student in electrical engineering and computer science and CSAIL affiliate who’s a lead author on a new paper about the approach. “We use domain-specific data to teach the base model about its counterpart’s expertise in areas like biomedical tasks and math and reasoning questions. This process automatically finds the parts of the data that are hard for the base model to generate, and then it instructs the base model to switch to the expert LLM, which was pretrained on data from a similar field. The general-purpose model provides the ‘scaffolding’ generation, and when it calls on the specialized LLM, it prompts the expert to generate the desired tokens. Our findings indicate that the LLMs learn patterns of collaboration organically, resembling how humans recognize when to call upon an expert to fill in the blanks.”
A combination of flexibility and factuality
Imagine asking a general-purpose LLM to name the ingredients of a specific prescription drug. It may reply incorrectly, necessitating the expertise of a specialized model.
To showcase Co-LLM’s flexibility, the researchers used data like the BioASQ medical set to couple a base LLM with expert LLMs in different domains, like the Meditron model, which is pretrained on unlabeled medical data. This enabled the algorithm to help answer inquiries a biomedical expert would typically receive, such as naming the mechanisms causing a particular disease.
For example, if you asked a simple LLM alone to name the ingredients of a specific prescription drug, it may reply incorrectly. With the added expertise of a model that specializes in biomedical data, you’d get a more accurate answer. Co-LLM also alerts users where to double-check answers.
Another example of Co-LLM’s performance boost: When tasked with solving a math problem like “a3 · a2 if a=5,” the general-purpose model incorrectly calculated the answer to be 125. As Co-LLM trained the model to collaborate more with a large math LLM called Llemma, together they determined that the correct solution was 3,125.
Co-LLM gave more accurate replies than fine-tuned simple LLMs and untuned specialized models working independently. Co-LLM can guide two models that were trained differently to work together, whereas other effective LLM collaboration approaches, such as “Proxy Tuning,” need all of their component models to be trained similarly. Additionally, this baseline requires each model to be used simultaneously to produce the answer, whereas MIT’s algorithm simply activates its expert model for particular tokens, leading to more efficient generation.
When to ask the expert
The MIT researchers’ algorithm highlights that imitating human teamwork more closely can increase accuracy in multi-LLM collaboration. To further elevate its factual precision, the team may draw from human self-correction: They’re considering a more robust deferral approach that can backtrack when the expert model doesn’t give a correct response. This upgrade would allow Co-LLM to course-correct so the algorithm can still give a satisfactory reply.
The team would also like to update the expert model (via only training the base model) when new information is available, keeping answers as current as possible. This would allow Co-LLM to pair the most up-to-date information with strong reasoning power. Eventually, the model could assist with enterprise documents, using the latest information it has to update them accordingly. Co-LLM could also train small, private models to work with a more powerful LLM to improve documents that must remain within the server.
“Co-LLM presents an interesting approach for learning to choose between two models to improve efficiency and performance,” says Colin Raffel, associate professor at the University of Toronto and an associate research director at the Vector Institute, who wasn’t involved in the research. “Since routing decisions are made at the token-level, Co-LLM provides a granular way of deferring difficult generation steps to a more powerful model. The unique combination of model-token-level routing also provides a great deal of flexibility that similar methods lack. Co-LLM contributes to an important line of work that aims to develop ecosystems of specialized models to outperform expensive monolithic AI systems.”
Shen wrote the paper with four other CSAIL affiliates: PhD student Hunter Lang ’17, MEng ’18; former postdoc and Apple AI/ML researcher Bailin Wang; MIT assistant professor of electrical engineering and computer science Yoon Kim, and professor and Jameel Clinic member David Sontag PhD ’10, who are both part of MIT-IBM Watson AI Lab. Their research was supported, in part, by the National Science Foundation, The National Defense Science and Engineering Graduate (NDSEG) Fellowship, MIT-IBM Watson AI Lab, and Amazon. Their work was presented at the Annual Meeting of the Association for Computational Linguistics.
Affordable high-tech windows for comfort and energy savings
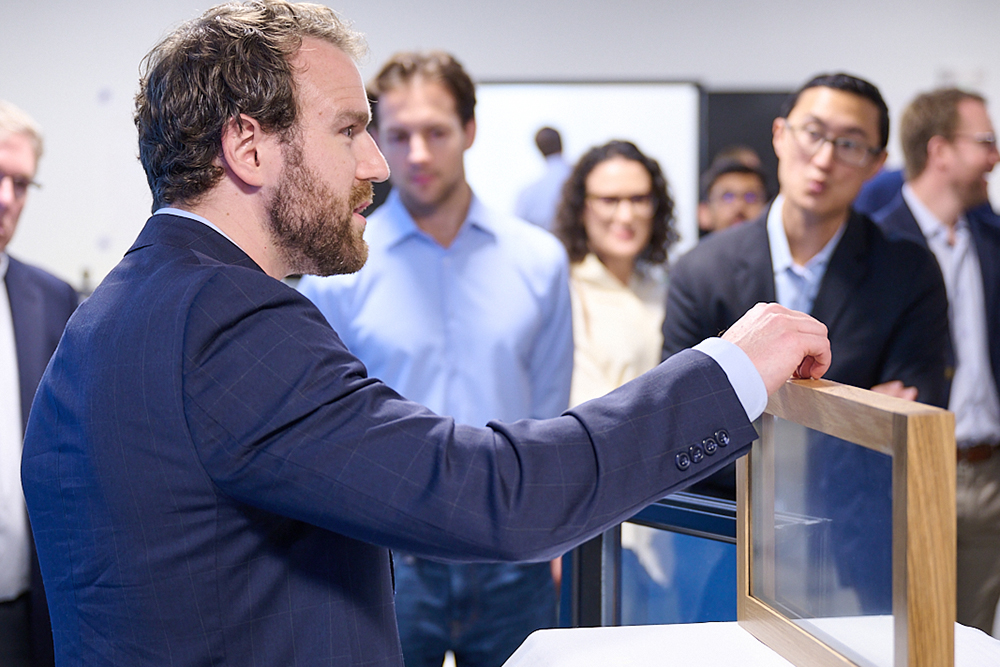
Imagine if the windows of your home didn’t transmit heat. They’d keep the heat indoors in winter and outdoors on a hot summer’s day. Your heating and cooling bills would go down; your energy consumption and carbon emissions would drop; and you’d still be comfortable all year ’round.
AeroShield, a startup spun out of MIT, is poised to start manufacturing such windows. Building operations make up 36 percent of global carbon dioxide emissions, and today’s windows are a major contributor to energy inefficiency in buildings. To improve building efficiency, AeroShield has developed a window technology that promises to reduce heat loss by up to 65 percent, significantly reducing energy use and carbon emissions in buildings, and the company just announced the opening of a new facility to manufacture its breakthrough energy-efficient windows.
“Our mission is to decarbonize the built environment,” says Elise Strobach SM ’17, PhD ’20, co-founder and CEO of AeroShield. “The availability of affordable, thermally insulating windows will help us achieve that goal while also reducing homeowner’s heating and cooling bills.” According to the U.S. Department of Energy, for most homeowners, 30 percent of that bill results from window inefficiencies.
Technology development at MIT
Research on AeroShield’s window technology began a decade ago in the MIT lab of Evelyn Wang, Ford Professor of Engineering, now on leave to serve as director of the Advanced Research Projects Agency-Energy (ARPA-E). In late 2014, the MIT team received funding from ARPA-E, and other sponsors followed, including the MIT Energy Initiative through the MIT Tata Center for Technology and Design in 2016.
The work focused on aerogels, remarkable materials that are ultra-porous, lighter than a marshmallow, strong enough to support a brick, and an unparalleled barrier to heat flow. Aerogels were invented in the 1930s and used by NASA and others as thermal insulation. The team at MIT saw the potential for incorporating aerogel sheets into windows to keep heat from escaping or entering buildings. But there was one problem: Nobody had been able to make aerogels transparent.
An aerogel is made of transparent, loosely connected nanoscale silica particles and is 95 percent air. But an aerogel sheet isn’t transparent because light traveling through it gets scattered by the silica particles.
After five years of theoretical and experimental work, the MIT team determined that the key to transparency was having the silica particles both small and uniform in size. This allows light to pass directly through, so the aerogel becomes transparent. Indeed, as long as the particle size is small and uniform, increasing the thickness of an aerogel sheet to achieve greater thermal insulation won’t make it less clear.
Teams in the MIT lab looked at various applications for their super-insulating, transparent aerogels. Some focused on improving solar thermal collectors by making the systems more efficient and less expensive. But to Strobach, increasing the thermal efficiency of windows looked especially promising and potentially significant as a means of reducing climate change.
The researchers determined that aerogel sheets could be inserted into the gap in double-pane windows, making them more than twice as insulating. The windows could then be manufactured on existing production lines with minor changes, and the resulting windows would be affordable and as wide-ranging in style as the window options available today. Best of all, once purchased and installed, the windows would reduce electricity bills, energy use, and carbon emissions.
The impact on energy use in buildings could be considerable. “If we only consider winter, windows in the United States lose enough energy to power over 50 million homes,” says Strobach. “That wasted energy generates about 350 million tons of carbon dioxide — more than is emitted by 76 million cars.” Super-insulating windows could help home and building owners reduce carbon dioxide emissions by gigatons while saving billions in heating and cooling costs.
The AeroShield story
In 2019, Strobach and her MIT colleagues — Aaron Baskerville-Bridges MBA ’20, SM ’20 and Kyle Wilke PhD ’19 — co-founded AeroShield to further develop and commercialize their aerogel-based technology for windows and other applications. And in the subsequent five years, their hard work has attracted attention, recently leading to two major accomplishments.
In spring 2024, the company announced the opening of its new pilot manufacturing facility in Waltham, Massachusetts, where the team will be producing, testing, and certifying their first full-size windows and patio doors for initial product launch. The 12,000 square foot facility will significantly expand the company’s capabilities, with cutting-edge aerogel R&D labs, manufacturing equipment, assembly lines, and testing equipment. Says Strobach, “Our pilot facility will supply window and door manufacturers as we launch our first products and will also serve as our R&D headquarters as we develop the next generation of energy-efficient products using transparent aerogels.”
Also in spring 2024, AeroShield received a $14.5 million award from ARPA-E’s “Seeding Critical Advances for Leading Energy technologies with Untapped Potential” (SCALEUP) program, which provides new funding to previous ARPA-E awardees that have “demonstrated a viable path to market.” That funding will enable the company to expand its production capacity to tens of thousands, or even hundreds of thousands, of units per year.
Strobach also cites two less-obvious benefits of the SCALEUP award.
First, the funding is enabling the company to move more quickly on the scale-up phase of their technology development. “We know from our fundamental studies and lab experiments that we can make large-area aerogel sheets that could go in an entry or patio door,” says Elise. “The SCALEUP award allows us to go straight for that vision. We don’t have to do all the incremental sizes of aerogels to prove that we can make a big one. The award provides capital for us to buy the big equipment to make the big aerogel.”
Second, the SCALEUP award confirms the viability of the company to other potential investors and collaborators. Indeed, AeroShield recently announced $5 million of additional funding from existing investors Massachusetts Clean Energy Center and MassVentures, as well as new investor MassMutual Ventures. Strobach notes that the company now has investor, engineering, and customer partners.
She stresses the importance of partners in achieving AeroShield’s mission. “We know that what we’ve got from a fundamental perspective can change the industry,” she says. “Now we want to go out and do it. With the right partners and at the right pace, we may actually be able to increase the energy efficiency of our buildings early enough to help make a real dent in climate change.”
Finding some stability in adaptable brains
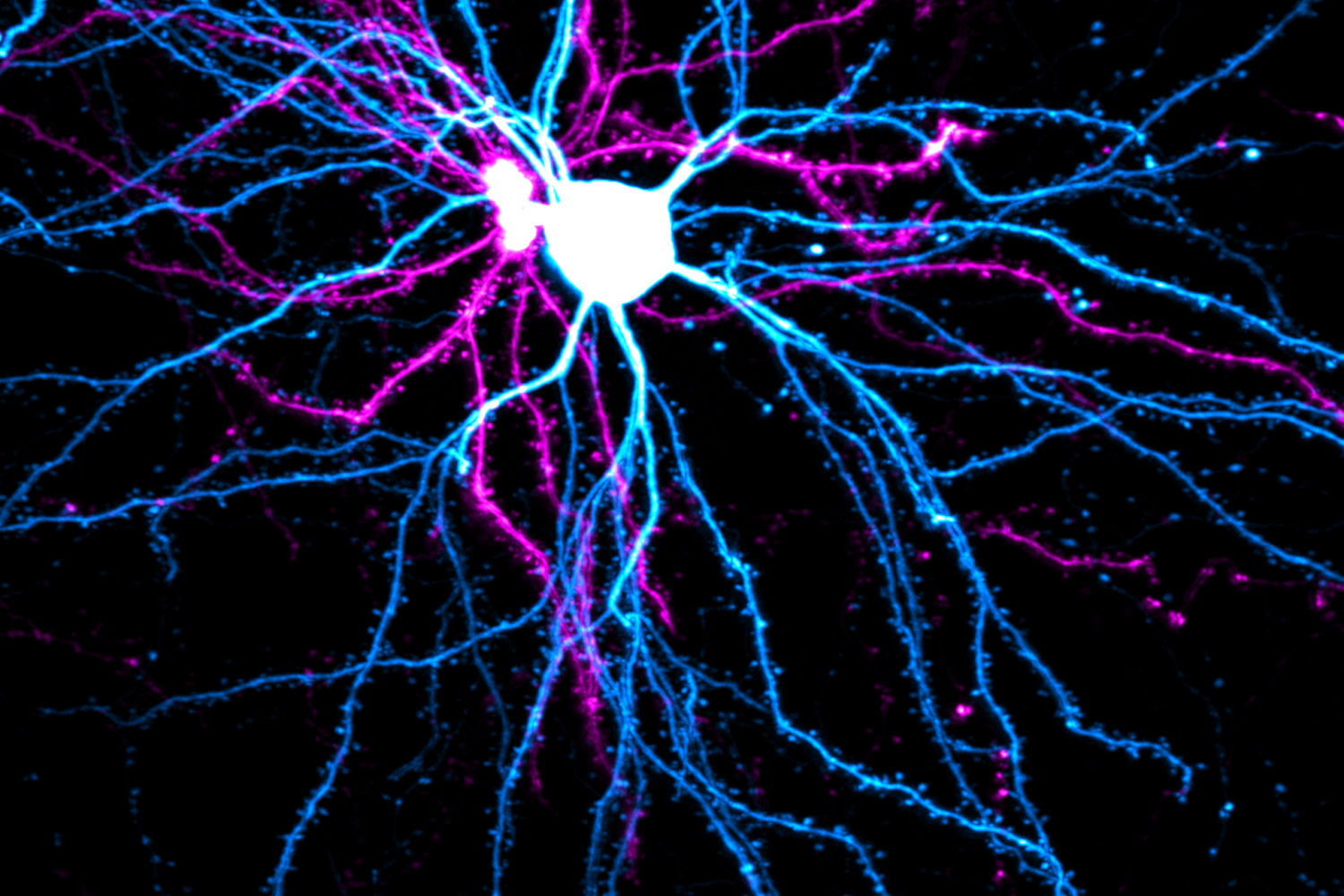
One of the brain’s most celebrated qualities is its adaptability. Changes to neural circuits, whose connections are continually adjusted as we experience and interact with the world, are key to how we learn. But to keep knowledge and memories intact, some parts of the circuitry must be resistant to this constant change.
“Brains have figured out how to navigate this landscape of balancing between stability and flexibility, so that you can have new learning and you can have lifelong memory,” says neuroscientist Mark Harnett, an investigator at MIT’s McGovern Institute for Brain Research. In the Aug. 27 issue of the journal Cell Reports, Harnett and his team show how individual neurons can contribute to both parts of this vital duality. By studying the synapses through which pyramidal neurons in the brain’s sensory cortex communicate, they have learned how the cells preserve their understanding of some of the world’s most fundamental features, while also maintaining the flexibility they need to adapt to a changing world.
Visual connections
Pyramidal neurons receive input from other neurons via thousands of connection points. Early in life, these synapses are extremely malleable; their strength can shift as a young animal takes in visual information and learns to interpret it. Most remain adaptable into adulthood, but Harnett’s team discovered that some of the cells’ synapses lose their flexibility when the animals are less than a month old. Having both stable and flexible synapses means these neurons can combine input from different sources to use visual information in flexible ways.
Postdoc Courtney Yaeger took a close look at these unusually stable synapses, which cluster together along a narrow region of the elaborately branched pyramidal cells. She was interested in the connections through which the cells receive primary visual information, so she traced their connections with neurons in a vision-processing center of the brain’s thalamus called the dorsal lateral geniculate nucleus (dLGN).
The long extensions through which a neuron receives signals from other cells are called dendrites, and they branch of from the main body of the cell into a tree-like structure. Spiny protrusions along the dendrites form the synapses that connect pyramidal neurons to other cells. Yaeger’s experiments showed that connections from the dLGN all led to a defined region of the pyramidal cells — a tight band within what she describes as the trunk of the dendritic tree.
Yaeger found several ways in which synapses in this region — formally known as the apical oblique dendrite domain — differ from other synapses on the same cells. “They’re not actually that far away from each other, but they have completely different properties,” she says.
Stable synapses
In one set of experiments, Yaeger activated synapses on the pyramidal neurons and measured the effect on the cells’ electrical potential. Changes to a neuron’s electrical potential generate the impulses the cells use to communicate with one another. It is common for a synapse’s electrical effects to amplify when synapses nearby are also activated. But when signals were delivered to the apical oblique dendrite domain, each one had the same effect, no matter how many synapses were stimulated. Synapses there don’t interact with one another at all, Harnett says. “They just do what they do. No matter what their neighbors are doing, they all just do kind of the same thing.”
The team was also able to visualize the molecular contents of individual synapses. This revealed a surprising lack of a certain kind of neurotransmitter receptor, called NMDA receptors, in the apical oblique dendrites. That was notable because of NMDA receptors’ role in mediating changes in the brain. “Generally when we think about any kind of learning and memory and plasticity, it’s NMDA receptors that do it,” Harnett says. “That is the by far most common substrate of learning and memory in all brains.”
When Yaeger stimulated the apical oblique synapses with electricity, generating patterns of activity that would strengthen most synapses, the team discovered a consequence of the limited presence of NMDA receptors. The synapses’ strength did not change. “There’s no activity-dependent plasticity going on there, as far as we have tested,” Yaeger says.
That makes sense, the researchers say, because the cells’ connections from the thalamus relay primary visual information detected by the eyes. It is through these connections that the brain learns to recognize basic visual features like shapes and lines.
“These synapses are basically a robust, high-fidelity readout of this visual information,” Harnett explains. “That’s what they’re conveying, and it’s not context-sensitive. So it doesn’t matter how many other synapses are active, they just do exactly what they’re going to do, and you can’t modify them up and down based on activity. So they’re very, very stable.”
“You actually don’t want those to be plastic,” adds Yaeger. “Can you imagine going to sleep and then forgetting what a vertical line looks like? That would be disastrous.”
By conducting the same experiments in mice of different ages, the researchers determined that the synapses that connect pyramidal neurons to the thalamus become stable a few weeks after young mice first open their eyes. By that point, Harnett says, they have learned everything they need to learn. On the other hand, if mice spend the first weeks of their lives in the dark, the synapses never stabilize — further evidence that the transition depends on visual experience.
The team’s findings not only help explain how the brain balances flexibility and stability; they could help researchers teach artificial intelligence how to do the same thing. Harnett says artificial neural networks are notoriously bad at this: when an artificial neural network that does something well is trained to do something new, it almost always experiences “catastrophic forgetting” and can no longer perform its original task. Harnett’s team is exploring how they can use what they’ve learned about real brains to overcome this problem in artificial networks.