OpenAI is awarding a $1 million grant to a Duke University research team to look at how AI could predict human moral judgments. The initiative highlights the growing focus on the intersection of technology and ethics, and raises critical questions: Can AI handle the complexities of…
Only 2.1% avoided generative AI in 2024: Find out why
This significant drop suggests various important underlying factors, like increased awareness and understanding, broader accessibility, and more….
How blockchain, IoT, and AI shape digital transformation
When devices, networks, and AI work together seamlessly, it creates a smarter, more connected ecosystem. This isn’t a distant dream; it’s a reality rapidly emerging as blockchain, IoT, and AI come together. These technologies are no longer working in isolation – they form a trio that…
US eyes AGI breakthrough in escalating China tech rivalry
The emerging US-China Artificial General Intelligence (AGI) rivalry could face a major policy transformation, as the US-China Economic and Security Review Commission (USCC) recommends a Manhattan Project-style initiative and restrictions on humanoid robots in its latest report to Congress. Released in November 2024, the Commission’s annual report…
Ordnance Survey: Navigating the role of AI and ethical considerations in geospatial technology – AI News
As we approach a new year filled with potential, the landscape of technology, particularly artificial intelligence (AI) and machine learning (ML), is on the brink of significant transformation. Manish Jethwa, CTO at Ordnance Survey (OS), the national mapping agency for Great Britain, offers an insightful glimpse…
Helping students bring about decarbonization, from benchtop to global energy marketplace
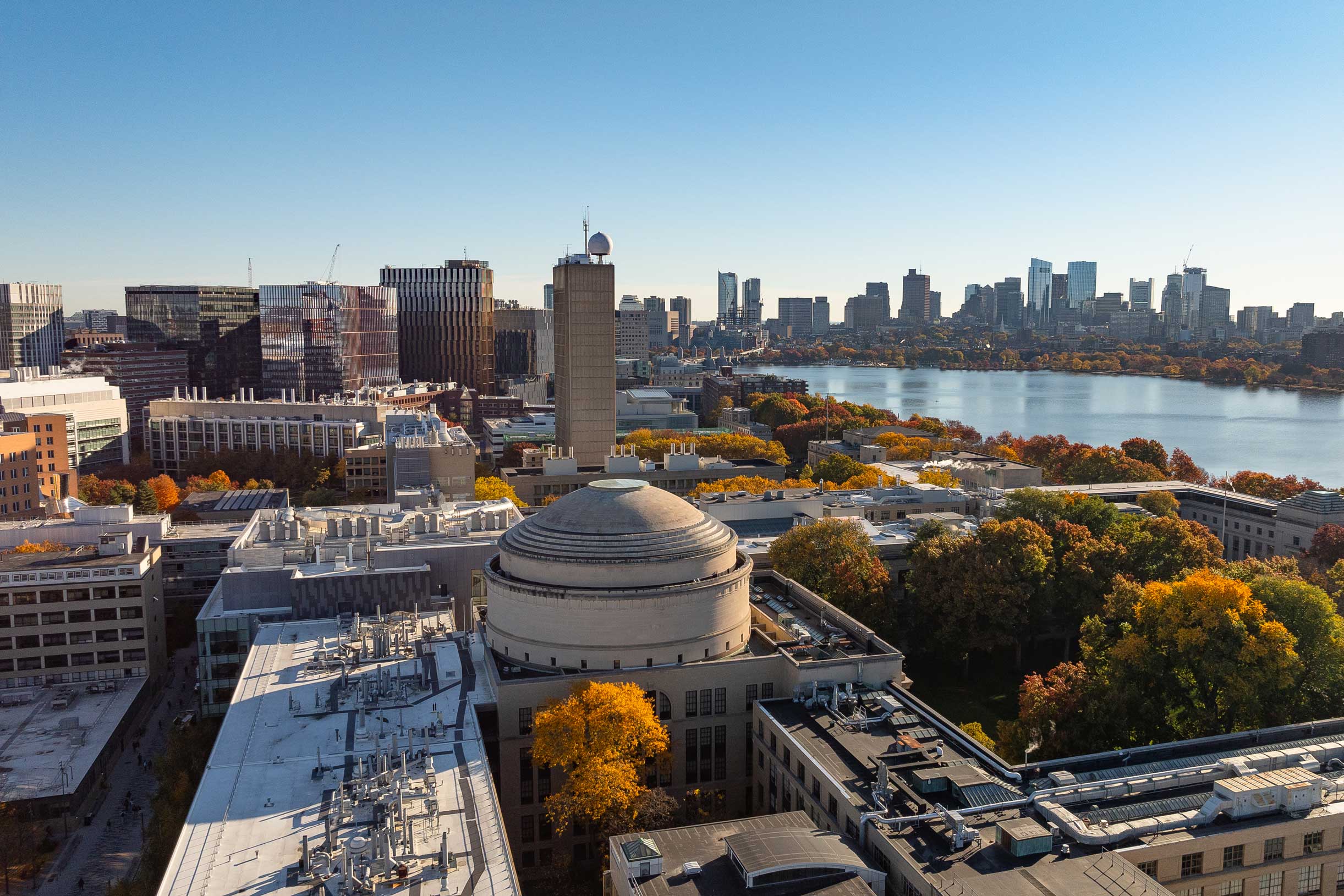
MIT students are adept at producing research and innovations at the cutting edge of their fields. But addressing a problem as large as climate change requires understanding the world’s energy landscape, as well as the ways energy technologies evolve over time.
Since 2010, the course IDS.521/IDS.065 (Energy Systems for Climate Change Mitigation) has equipped students with the skills they need to evaluate the various energy decarbonization pathways available to the world. The work is designed to help them maximize their impact on the world’s emissions by making better decisions along their respective career paths.
“The question guiding my teaching and research is how do we solve big societal challenges with technology, and how can we be more deliberate in developing and supporting technologies to get us there?” says Professor Jessika Trancik, who started the course to help fill a gap in knowledge about the ways technologies evolve and scale over time.
Since its inception in 2010, the course has attracted graduate students from across MIT’s five schools. The course has also recently opened to undergraduate students and been adapted to an online course for professionals.
Class sessions alternate between lectures and student discussions that lead up to semester-long projects in which groups of students explore specific strategies and technologies for reducing global emissions. This year’s projects span several topics, including how quickly transmission infrastructure is expanding, the relationship between carbon emissions and human development, and how to decarbonize the production of key chemicals.
The curriculum is designed to help students identify the most promising ways to mitigate climate change whether they plan to be scientists, engineers, policymakers, investors, urban planners, or just more informed citizens.
“We’re coming at this issue from both sides,” explains Trancik, who is part of MIT’s Institute for Data, Systems, and Society. “Engineers are used to designing a technology to work as well as possible here and now, but not always thinking over a longer time horizon about a technology evolving and succeeding in the global marketplace. On the flip side, for students at the macro level, often studies in policy and economics of technological change don’t fully account for the physical and engineering constraints of rates of improvement. But all of that information allows you to make better decisions.”
Bridging the gap
As a young researcher working on low-carbon polymers and electrode materials for solar cells, Trancik always wondered how the materials she worked on would scale in the real world. They might achieve promising performance benchmarks in the lab, but would they actually make a difference in mitigating climate change? Later, she began focusing increasingly on developing methods for predicting how technologies might evolve.
“I’ve always been interested in both the macro and the micro, or even nano, scales,” Trancik says. “I wanted to know how to bridge these new technologies we’re working on with the big picture of where we want to go.”
Trancik’ described her technology-grounded approach to decarbonization in a paper that formed the basis for IDS.065. In the paper, she presented a way to evaluate energy technologies against climate-change mitigation goals while focusing on the technology’s evolution.
“That was a departure from previous approaches, which said, given these technologies with fixed characteristics and assumptions about their rates of change, how do I choose the best combination?” Trancik explains. “Instead we asked: Given a goal, how do we develop the best technologies to meet that goal? That inverts the problem in a way that’s useful to engineers developing these technologies, but also to policymakers and investors that want to use the evolution of technologies as a tool for achieving their objectives.”
This past semester, the class took place every Tuesday and Thursday in a classroom on the first floor of the Stata Center. Students regularly led discussions where they reflected on the week’s readings and offered their own insights.
“Students always share their takeaways and get to ask open questions of the class,” says Megan Herrington, a PhD candidate in the Department of Chemical Engineering. “It helps you understand the readings on a deeper level because people with different backgrounds get to share their perspectives on the same questions and problems. Everybody comes to class with their own lens, and the class is set up to highlight those differences.”
The semester begins with an overview of climate science, the origins of emissions reductions goals, and technology’s role in achieving those goals. Students then learn how to evaluate technologies against decarbonization goals.
But technologies aren’t static, and neither is the world. Later lessons help students account for the change of technologies over time, identifying the mechanisms for that change and even forecasting rates of change.
Students also learn about the role of government policy. This year, Trancik shared her experience traveling to the COP29 United Nations Climate Change Conference.
“It’s not just about technology,” Trancik says. “It’s also about the behaviors that we engage in and the choices we make. But technology plays a major role in determining what set of choices we can make.”
From the classroom to the world
Students in the class say it has given them a new perspective on climate change mitigation.
“I have really enjoyed getting to see beyond the research people are doing at the benchtop,” says Herrington. “It’s interesting to see how certain materials or technologies that aren’t scalable yet may fit into a larger transformation in energy delivery and consumption. It’s also been interesting to pull back the curtain on energy systems analysis to understand where the metrics we cite in energy-related research originate from, and to anticipate trajectories of emerging technologies.”
Onur Talu, a first-year master’s student in the Technology and Policy Program, says the class has made him more hopeful.
“I came into this fairly pessimistic about the climate,” says Talu, who has worked for clean technology startups in the past. “This class has taught me different ways to look at the problem of climate change mitigation and developing renewable technologies. It’s also helped put into perspective how much we’ve accomplished so far.”
Several student projects from the class over the years have been developed into papers published in peer-reviewed journals. They have also been turned into tools, like carboncounter.com, which plots the emissions and costs of cars and has been featured in The New York Times.
Former class students have also launched startups; Joel Jean SM ’13, PhD ’17, for example, started Swift Solar. Others have drawn on the course material to develop impactful careers in government and academia, such as Patrick Brown PhD ’16 at the National Renewable Energy Laboratory and Leah Stokes SM ’15, PhD ’15 at the University of California at Santa Barbara.
Overall, students say the course helps them take a more informed approach to applying their skills toward addressing climate change.
“It’s not enough to just know how bad climate change could be,” says Yu Tong, a first-year master’s student in civil and environmental engineering. “It’s also important to understand how technology can work to mitigate climate change from both a technological and market perspective. It’s about employing technology to solve these issues rather than just working in a vacuum.”
The Race for AI Reasoning is Challenging our Imagination
New reasoning models from Google and OpenAI…
Sora AI Review: Will AI Replace Videographers For Good?
Have you ever wanted to create high-quality videos from nothing but words? In February 2024, OpenAI unveiled Sora, an AI system capable of creating photorealistic videos from text prompts that can be up to 20 seconds long. Since December 2024, the tool has been accessible to…
Ecologists find computer vision models’ blind spots in retrieving wildlife images
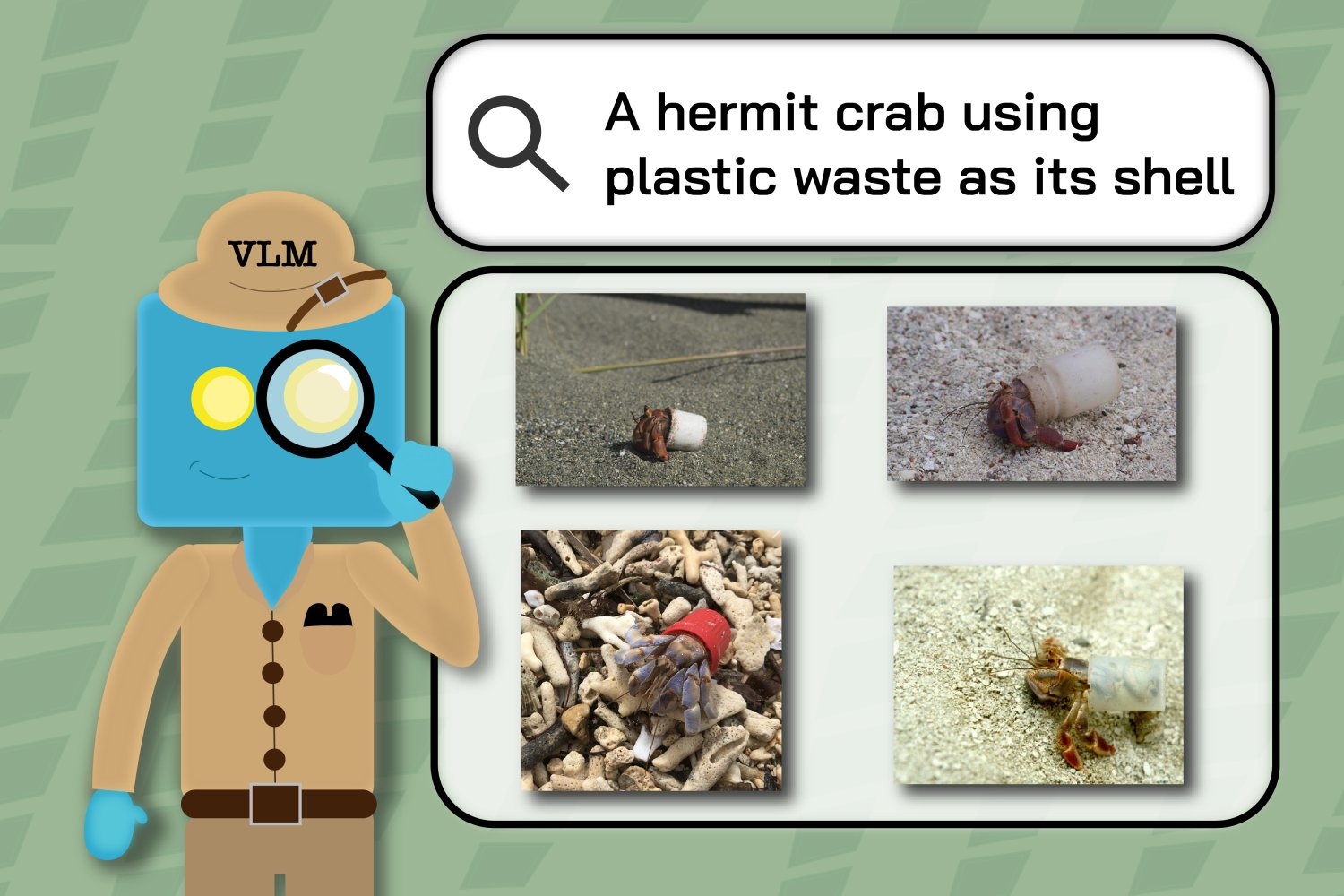
Try taking a picture of each of North America’s roughly 11,000 tree species, and you’ll have a mere fraction of the millions of photos within nature image datasets. These massive collections of snapshots — ranging from butterflies to humpback whales — are a great research tool for ecologists because they provide evidence of organisms’ unique behaviors, rare conditions, migration patterns, and responses to pollution and other forms of climate change.
While comprehensive, nature image datasets aren’t yet as useful as they could be. It’s time-consuming to search these databases and retrieve the images most relevant to your hypothesis. You’d be better off with an automated research assistant — or perhaps artificial intelligence systems called multimodal vision language models (VLMs). They’re trained on both text and images, making it easier for them to pinpoint finer details, like the specific trees in the background of a photo.
But just how well can VLMs assist nature researchers with image retrieval? A team from MIT’s Computer Science and Artificial Intelligence Laboratory (CSAIL), University College London, iNaturalist, and elsewhere designed a performance test to find out. Each VLM’s task: locate and reorganize the most relevant results within the team’s “INQUIRE” dataset, composed of 5 million wildlife pictures and 250 search prompts from ecologists and other biodiversity experts.
Looking for that special frog
In these evaluations, the researchers found that larger, more advanced VLMs, which are trained on far more data, can sometimes get researchers the results they want to see. The models performed reasonably well on straightforward queries about visual content, like identifying debris on a reef, but struggled significantly with queries requiring expert knowledge, like identifying specific biological conditions or behaviors. For example, VLMs somewhat easily uncovered examples of jellyfish on the beach, but struggled with more technical prompts like “axanthism in a green frog,” a condition that limits their ability to make their skin yellow.
Their findings indicate that the models need much more domain-specific training data to process difficult queries. MIT PhD student Edward Vendrow, a CSAIL affiliate who co-led work on the dataset in a new paper, believes that by familiarizing with more informative data, the VLMs could one day be great research assistants. “We want to build retrieval systems that find the exact results scientists seek when monitoring biodiversity and analyzing climate change,” says Vendrow. “Multimodal models don’t quite understand more complex scientific language yet, but we believe that INQUIRE will be an important benchmark for tracking how they improve in comprehending scientific terminology and ultimately helping researchers automatically find the exact images they need.”
The team’s experiments illustrated that larger models tended to be more effective for both simpler and more intricate searches due to their expansive training data. They first used the INQUIRE dataset to test if VLMs could narrow a pool of 5 million images to the top 100 most-relevant results (also known as “ranking”). For straightforward search queries like “a reef with manmade structures and debris,” relatively large models like “SigLIP” found matching images, while smaller-sized CLIP models struggled. According to Vendrow, larger VLMs are “only starting to be useful” at ranking tougher queries.
Vendrow and his colleagues also evaluated how well multimodal models could re-rank those 100 results, reorganizing which images were most pertinent to a search. In these tests, even huge LLMs trained on more curated data, like GPT-4o, struggled: Its precision score was only 59.6 percent, the highest score achieved by any model.
The researchers presented these results at the Conference on Neural Information Processing Systems (NeurIPS) earlier this month.
Inquiring for INQUIRE
The INQUIRE dataset includes search queries based on discussions with ecologists, biologists, oceanographers, and other experts about the types of images they’d look for, including animals’ unique physical conditions and behaviors. A team of annotators then spent 180 hours searching the iNaturalist dataset with these prompts, carefully combing through roughly 200,000 results to label 33,000 matches that fit the prompts.
For instance, the annotators used queries like “a hermit crab using plastic waste as its shell” and “a California condor tagged with a green ‘26’” to identify the subsets of the larger image dataset that depict these specific, rare events.
Then, the researchers used the same search queries to see how well VLMs could retrieve iNaturalist images. The annotators’ labels revealed when the models struggled to understand scientists’ keywords, as their results included images previously tagged as irrelevant to the search. For example, VLMs’ results for “redwood trees with fire scars” sometimes included images of trees without any markings.
“This is careful curation of data, with a focus on capturing real examples of scientific inquiries across research areas in ecology and environmental science,” says Sara Beery, the Homer A. Burnell Career Development Assistant Professor at MIT, CSAIL principal investigator, and co-senior author of the work. “It’s proved vital to expanding our understanding of the current capabilities of VLMs in these potentially impactful scientific settings. It has also outlined gaps in current research that we can now work to address, particularly for complex compositional queries, technical terminology, and the fine-grained, subtle differences that delineate categories of interest for our collaborators.”
“Our findings imply that some vision models are already precise enough to aid wildlife scientists with retrieving some images, but many tasks are still too difficult for even the largest, best-performing models,” says Vendrow. “Although INQUIRE is focused on ecology and biodiversity monitoring, the wide variety of its queries means that VLMs that perform well on INQUIRE are likely to excel at analyzing large image collections in other observation-intensive fields.”
Inquiring minds want to see
Taking their project further, the researchers are working with iNaturalist to develop a query system to better help scientists and other curious minds find the images they actually want to see. Their working demo allows users to filter searches by species, enabling quicker discovery of relevant results like, say, the diverse eye colors of cats. Vendrow and co-lead author Omiros Pantazis, who recently received his PhD from University College London, also aim to improve the re-ranking system by augmenting current models to provide better results.
University of Pittsburgh Associate Professor Justin Kitzes highlights INQUIRE’s ability to uncover secondary data. “Biodiversity datasets are rapidly becoming too large for any individual scientist to review,” says Kitzes, who wasn’t involved in the research. “This paper draws attention to a difficult and unsolved problem, which is how to effectively search through such data with questions that go beyond simply ‘who is here’ to ask instead about individual characteristics, behavior, and species interactions. Being able to efficiently and accurately uncover these more complex phenomena in biodiversity image data will be critical to fundamental science and real-world impacts in ecology and conservation.”
Vendrow, Pantazis, and Beery wrote the paper with iNaturalist software engineer Alexander Shepard, University College London professors Gabriel Brostow and Kate Jones, University of Edinburgh associate professor and co-senior author Oisin Mac Aodha, and University of Massachusetts at Amherst Assistant Professor Grant Van Horn, who served as co-senior author. Their work was supported, in part, by the Generative AI Laboratory at the University of Edinburgh, the U.S. National Science Foundation/Natural Sciences and Engineering Research Council of Canada Global Center on AI and Biodiversity Change, a Royal Society Research Grant, and the Biome Health Project funded by the World Wildlife Fund United Kingdom.
Hunyuan-Large and the MoE Revolution: How AI Models Are Growing Smarter and Faster
Artificial Intelligence (AI) is advancing at an extraordinary pace. What seemed like a futuristic concept just a decade ago is now part of our daily lives. However, the AI we encounter now is only the beginning. The fundamental transformation is yet to be witnessed due to…