As the EU’s AI Act prepares to come into force tomorrow, industry experts are weighing in on its potential impact, highlighting its role in building trust and encouraging responsible AI adoption. Curtis Wilson, Staff Data Engineer at Synopsys’ Software Integrity Group, believes the new regulation could…
Apple opts for Google chips in AI infrastructure, sidestepping Nvidia
In a report published on Monday, it was disclosed that Apple sidestepped industry leader Nvidia in favour of chips designed by Google. Instead of employing Nvidia’s GPUs for its artificial intelligence software infrastructure, Apple will use Google chips as the cornerstone of AI-related features and tools…
UAE blocks US congressional meetings with G42 amid AI transfer concerns
There have been reports that the United Arab Emirates (UAE) has “suddenly cancelled” the ongoing series of meetings between a group of US congressional staffers and Emirati AI firm G42, after some US lawmakers raised concerns that this practice may lead to the transfer of advanced…
Canva Expands AI Capabilities with Acquisition of Leonardo.ai
Canva has announced its acquisition of Leonardo.ai, an Australian generative AI startup. This strategic purchase positions Canva to compete more aggressively in the rapidly evolving market for AI-enhanced design platforms. The acquisition of Leonardo.ai represents a major step forward for Canva in its quest to build…
Testing AI Tools? Don’t Forget to Think About the Total Cost.
In 2023, AI quickly moved from a novel and futuristic idea to a core component of enterprise strategies everywhere. While ChatGPT is one of the most popular shadow IT software applications, IT leaders are already working to formally adopt AI tools. While the average use of…
8 CSS & JavaScript Snippets for Creating Animated Progress Bars – Speckyboy
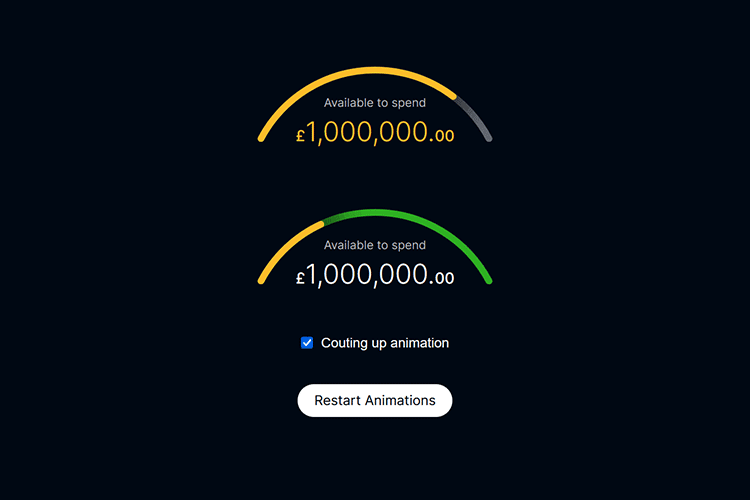
User interfaces (UIs) that measure progress are helpful. They offer visual confirmation when completing various tasks, so users don’t have to guess how far they are into a process.
We see these UIs on our devices. Anyone who’s performed an update on their computer or phone will be familiar with them. Thus, it’s easy to take their design for granted.
However, we’re starting to see more creative implementations. And the web has become a driving force. Designers are using CSS and JavaScript to make progress UIs fun and informative. By adding quirky animations and other visuals, we’re well beyond the standard progress bar.
Here are eight progress bars and UIs that have something unique to offer. You might be surprised at how far these elements have come.
Animated Semi-Circular Progress Bar Chart Using SVG by Andrew Sims
We don’t always measure progress in a straight line. You can also use shapes like this beautiful semicircle. The snippet uses ProgressBar.js and SVG to create an attractive presentation.
See the Pen Animated semicircular progress bar chart using SVG by Andrew Sims
CSS Animated Download & Progress Animation by Aaron Iker
Users spend a lot of time downloading files. Progress meters keep them abreast of their status. We love that this example keeps things simple. A single button houses all the information users need.
See the Pen Download progress animation by Aaron Iker
Progress Bar Animation by Eva Wythien
Who says progress bars have to be boring? Here’s a look at how creativity can spice things up. CSS keyframe animations, patterns, and gradients add fun to the mix.
See the Pen Progress bar animation by Eva Wythien
CSS & JavaScript Progress Clock by Jon Kantner
Time is another way to measure progress, and this clock does so in a unique way. Hover on the date, hours, minutes, and seconds to focus on their meters. The effect takes a complex UI and breaks it into bite-sized chunks.
See the Pen Progress Clock by Jon Kantner
CSS-Only Order Process Steps by Jamie Coulter
Here’s a fun way to show users the steps in an eCommerce process. Clicking on a step reveals more details. Notice how the box icon changes along the way. This UI demonstrates progress and doubles as an onboarding component.
See the Pen CSS only order process steps by Jamie Coulter
Screen Wraparound Progress Bar by Thomas Vaeth
Progress UIs can also be scroll-based. In this case, a colored bar wraps around the viewport as you scroll. The effect goes in reverse as you move back to the top. Perhaps this example isn’t a fit for every use case. But it could be a companion to a storytelling website.
See the Pen Wraparound Progress Bar by Thomas Vaeth
Responsive Circle Progress Bar by Tigran Sargsyan
This snippet uses an HTML range input that syncs with a circular progress UI. The shape makes this one stand out. But so does the color-changing effect. As the slider value changes, so do the colors.
See the Pen Circle progress by Tigran Sargsyan
Rotating 3d Progress Bar by Amit
Here’s something different. These rotating 3D progress bars provide a futuristic look. That aside, they were built entirely with CSS, and they’re sure to draw attention.
See the Pen 3d progress bar v2 by Amit
Better Progress Through Code
There’s no reason to settle for an old-school progress UI. It’s now possible to create something that matches your desired aesthetic. And best of all, you don’t need a lot of complex code or imagery. Make these elements as simple or complex as you like.
The examples above demonstrate a wide range of possibilities. But they’re only scratching the surface. Combine CSS, JavaScript, and imagination to build a distinct look and feel.
Are you looking for more progress UI examples? You’ll want to check out our CodePen collection!
Related Topics
Top
Global data breach costs hit all-time high – CyberTalk
EXECUTIVE SUMMARY:
Global data breach costs have hit an all-time high, according to IBM’s annual Cost of a Data Breach report. The tech giant collaborated with the Ponemon institute to study more than 600 organizational breaches between March of 2023 and February of 2024.
The breaches affected 17 industries, across 16 countries and regions, and involved leaks of 2,000-113,000 records per breach. Here’s what researchers found…
Essential information
The global average cost of a data breach is $4.88 million, up nearly 10% from last year’s $4.5 million. Key drivers of the year-over-year cost spike included post-breach third-party expenses, along with lost business.
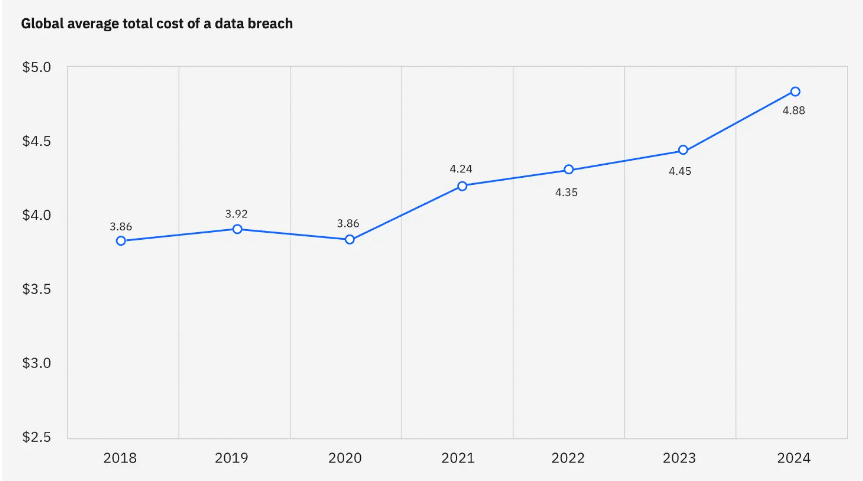
Over 50% of organizations that were interviewed said that they are passing the breach costs on to customers through higher prices for goods and services.
More key findings
- For the 14th consecutive year, the U.S. has the highest average data breach costs worldwide; nearly $9.4 million.
- In the last year, Canada and Japan both experienced drops in average breach costs.
- Most breaches could be traced back to one of two sources – stolen credentials or a phishing email.
- Seventy percent of organizations noted that breaches led to “significant” or “very significant” levels of disruption.
Deep-dive insights: AI
The report also observed that an increasing number of organizations are adopting artificial intelligence and automation to prevent breaches. Nearly two-thirds of organizations were found to have deployed AI and automation technologies across security operations centers.
The use of AI prevention workflows reduced the average cost of a breach by $2.2 million. Organizations without AI prevention workflows did not experience these cost savings.
Right now, only 20% of organizations report using gen AI security tools. However, those that have implemented them note a net positive effect. GenAI security tools can mitigate the average cost of a breach by more than $167,000, according to the report.
Deep-dive insights: Cloud
Multi-environment cloud breaches were found to cost more than $5 million to contend with, on average. Out of all breach types, they also took the longest time to identify and contain, reflecting the challenge that is identifying data and protecting it.
In regards to cloud-based breaches, commonly stolen data types included personal identifying information (PII) and intellectual property (IP).
As generative AI initiatives draw this data into new programs and processes, cyber security professionals are encouraged to reassess corresponding security and access controls.
The role of staffing issues
A number of organizations that contended with cyber attacks were found to have under-staffed cyber security teams. Staffing shortages are up 26% compared to last year.
Organizations with cyber security staff shortages averaged an additional $1.76 million in breach costs as compared to organizations with minimal or no staffing issues.
Staffing issues may be contributing to the increased use of AI and automation, which again, have been shown to reduce breach costs.
Further information
For more AI and cloud insights, click here. Access the Cost of a Data Breach 2024 report here. Lastly, to receive cyber security thought leadership articles, groundbreaking research and emerging threat analyses each week, subscribe to the CyberTalk.org newsletter.
The Casting of Frank Stone Preview – Breaking Down The Game’s Cutting Room Floor Feature And Scope Of Choice – Game Informer
The Casting of Frank Stone may have new elements due to its ties to Dead by Daylight, but it remains a Supermassive horror game at its core. By that, I mean it’s a narrative-focused, choice-driven adventure that can result in numerous different outcomes based on your decisions and reaction time to sudden button prompts. Characters can be permanently killed off due to your actions, and this blueprint has given past Supermassive works like Until Dawn and especially The Quarry (which boasted 186 different outcomes) plenty of replayability for fans who wanted to see every possible route the story could take. This has typically meant restarting the entire game, but The Casting of Frank Stone eases this process thanks to a new destination called the Cutting Room Floor.
This mode opens after you’ve beaten the game once, but it will be available at the start for owners of the Deluxe Edition. The Cutting Room Floor displays the web of possible outcomes, locked and unlocked, for every narrative fork in each chapter. It also shows the number of collectibles you’ve found or can be found.
Every decision has a percentage number representing the number of players who chose it, and this statistic will continually fluctuate as more people play. You can replay any segment, which means you can preserve your choices from a previous section of the game and only change later outcomes, and vice versa. Since some outcomes can only be experienced by making a specific combination of decisions, the Cutting Room Floor seems like a great, streamlined way to witness the different story permutations and go collectible/achievement hunting without replaying unnecessary stretches or the whole game.
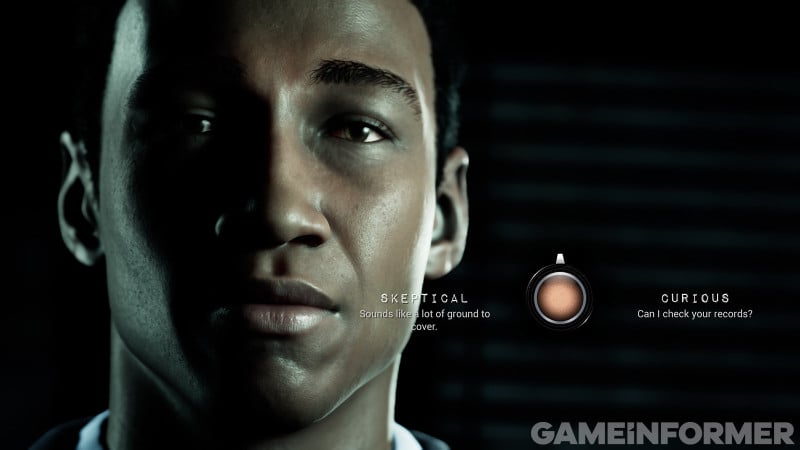
How many different directions can the story take? When I asked Supermassive Games this question, creative director Steve Goss told me that the sheer number of outcomes won’t be as vast as The Quarry’s. Instead, he says to compare the game to Until Dawn’s structure. The team aimed to write a more tightly written tale for The Casting of Frank Stone to facilitate more satisfying character arcs and resolutions. That said, you’ll still be making plenty of decisions, and the Cutting Room Floor will make it easier than ever to revisit those choices and make new ones.
The Casting of Frank Stone launches on September 3 for PlayStation 5, Xbox Series X/S, and PC. Click the banner below to visit our cover story hub for more exclusive stories and videos.
Mistral Large 2: Enhanced Code Generation and Multilingual Capabilities
Mistral AI introduced Mistral Large 2 on July 24, 2024. This latest model is a significant advancement in Artificial Intelligence (AI), providing extensive support for both programming and natural languages. Designed to handle complex tasks with greater accuracy and efficiency, Mistral Large 2 supports over 80…
Chaim Linhart, PhD, Co-founder & CTO of Ibex Medical Analytics – Interview Series
Chaim Linhart, PhD is the CTO and Co-Founder of Ibex Medical Analytics. He has more than 25 years of experience in algorithm development, AI and machine learning from academia as well as serving in an elite unit in the Israeli military and at several tech companies….