Videography Basics Everyone Needs to Know Well maybe not “everyone needs to know” but at least a few of us! So if you are using a consumer grade camera or a smartphone to capture your footage and you are just using the auto settings then you…
From steel engineering to ovarian tumor research
Ashutosh Kumar is a classically trained materials engineer. Having grown up with a passion for making things, he has explored steel design and studied stress fractures in alloys.
Throughout Kumar’s education, however, he was also drawn to biology and medicine. When he was accepted into an undergraduate metallurgical engineering and materials science program at Indian Institute of Technology (IIT) Bombay, the native of Jamshedpur was very excited — and “a little dissatisfied, since I couldn’t do biology anymore.”
Now a PhD candidate and a MathWorks Fellow in MIT’s Department of Materials Science and Engineering, Kumar can merge his wide-ranging interests. He studies the effect of certain bacteria that have been observed encouraging the spread of ovarian cancer and possibly reducing the effectiveness of chemotherapy and immunotherapy.
“Some microbes have an affinity toward infecting ovarian cancer cells, which can lead to changes in the cellular structure and reprogramming cells to survive in stressful conditions,” Kumar says. “This means that cells can migrate to different sites and may have a mechanism to develop chemoresistance. This opens an avenue to develop therapies to see if we can start to undo some of these changes.”
Kumar’s research combines microbiology, bioengineering, artificial intelligence, big data, and materials science. Using microbiome sequencing and AI, he aims to define microbiome changes that may correlate with poor patient outcomes. Ultimately, his goal is to engineer bacteriophage viruses to reprogram bacteria to work therapeutically.
Kumar started inching toward work in the health sciences just months into earning his bachelor’s degree at IIT Bombay.
“I realized engineering is so flexible that its applications extend to any field,” he says, adding that he started working with biomaterials “to respect both my degree program and my interests.”
“I loved it so much that I decided to go to graduate school,” he adds.
Starting his PhD program at MIT, he says, “was a fantastic opportunity to switch gears and work on more interdisciplinary or ‘MIT-type’ work.”
Kumar says he and Angela Belcher, the James Mason Crafts Professor of biological engineering and materials science, began discussing the impact of the microbiome on ovarian cancer when he first arrived at MIT.
“I shared my enthusiasm about human health and biology, and we started brainstorming,” he says. “We realized that there’s an unmet need to understand a lot of gynecological cancers. Ovarian cancer is an aggressive cancer, which is usually diagnosed when it’s too late and has already spread.”
In 2022, Kumar was awarded a MathWorks Fellowship. The fellowships are awarded to School of Engineering graduate students, preferably those who use MATLAB or Simulink — which were developed by the mathematical computer software company MathWorks — in their research. The philanthropic support fueled Kumar’s full transition into health science research.
“The work we are doing now was initially not funded by traditional sources, and the MathWorks Fellowship gave us the flexibility to pursue this field,” Kumar says. “It provided me with opportunities to learn new skills and ask questions about this topic. MathWorks gave me a chance to explore my interests and helped me navigate from being a steel engineer to a cancer scientist.”
Kumar’s work on the relationship between bacteria and ovarian cancer started with studying which bacteria are incorporated into tumors in mouse models.
“We started looking closely at changes in cell structure and how those changes impact cancer progression,” he says, adding that MATLAB image processing helps him and his collaborators track tumor metastasis.
The research team also uses RNA sequencing and MATLAB algorithms to construct a taxonomy of the bacteria.
“Once we have identified the microbiome composition,” Kumar says, “we want to see how the microbiome changes as cancer progresses and identify changes in, let’s say, patients who develop chemoresistance.”
He says recent findings that ovarian cancer may originate in the fallopian tubes are promising because detecting cancer-related biomarkers or lesions before cancer spreads to the ovaries could lead to better prognoses.
As he pursues his research, Kumar says he is extremely thankful to Belcher “for believing in me to work on this project.
“She trusted me and my passion for making an impact on human health — even though I come from a materials engineering background — and supported me throughout. It was her passion to take on new challenges that made it possible for me to work on this idea. She has been an amazing mentor and motivated me to continue moving forward.”
For her part, Belcher is equally enthralled.
“It has been amazing to work with Ashutosh on this ovarian cancer microbiome project,” she says. “He has been so passionate and dedicated to looking for less-conventional approaches to solve this debilitating disease. His innovations around looking for very early changes in the microenvironment of this disease could be critical in interception and prevention of ovarian cancer. We started this project with very little preliminary data, so his MathWorks fellowship was critical in the initiation of the project.”
Kumar, who has been very active in student government and community-building activities, believes it is very important for students to feel included and at home at their institutions so they can develop in ways outside of academics. He says that his own involvement helps him take time off from work.
“Science can never stop, and there will always be something to do,” he says, explaining that he deliberately schedules time off and that social engagement helps him to experience downtime. “Engaging with community members through events on campus or at the dorm helps set a mental boundary with work.”
Regarding his unusual route through materials science to cancer research, Kumar regards it as something that occurred organically.
“I have observed that life is very dynamic,” he says. “What we think we might do versus what we end up doing is never consistent. Five years back, I had no idea I would be at MIT working with such excellent scientific mentors around me.”
A better way to control shape-shifting soft robots
Imagine a slime-like robot that can seamlessly change its shape to squeeze through narrow spaces, which could be deployed inside the human body to remove an unwanted item.
While such a robot does not yet exist outside a laboratory, researchers are working to develop reconfigurable soft robots for applications in health care, wearable devices, and industrial systems.
But how can one control a squishy robot that doesn’t have joints, limbs, or fingers that can be manipulated, and instead can drastically alter its entire shape at will? MIT researchers are working to answer that question.
They developed a control algorithm that can autonomously learn how to move, stretch, and shape a reconfigurable robot to complete a specific task, even when that task requires the robot to change its morphology multiple times. The team also built a simulator to test control algorithms for deformable soft robots on a series of challenging, shape-changing tasks.
Their method completed each of the eight tasks they evaluated while outperforming other algorithms. The technique worked especially well on multifaceted tasks. For instance, in one test, the robot had to reduce its height while growing two tiny legs to squeeze through a narrow pipe, and then un-grow those legs and extend its torso to open the pipe’s lid.
While reconfigurable soft robots are still in their infancy, such a technique could someday enable general-purpose robots that can adapt their shapes to accomplish diverse tasks.
“When people think about soft robots, they tend to think about robots that are elastic, but return to their original shape. Our robot is like slime and can actually change its morphology. It is very striking that our method worked so well because we are dealing with something very new,” says Boyuan Chen, an electrical engineering and computer science (EECS) graduate student and co-author of a paper on this approach.
Chen’s co-authors include lead author Suning Huang, an undergraduate student at Tsinghua University in China who completed this work while a visiting student at MIT; Huazhe Xu, an assistant professor at Tsinghua University; and senior author Vincent Sitzmann, an assistant professor of EECS at MIT who leads the Scene Representation Group in the Computer Science and Artificial Intelligence Laboratory. The research will be presented at the International Conference on Learning Representations.
Controlling dynamic motion
Scientists often teach robots to complete tasks using a machine-learning approach known as reinforcement learning, which is a trial-and-error process in which the robot is rewarded for actions that move it closer to a goal.
This can be effective when the robot’s moving parts are consistent and well-defined, like a gripper with three fingers. With a robotic gripper, a reinforcement learning algorithm might move one finger slightly, learning by trial and error whether that motion earns it a reward. Then it would move on to the next finger, and so on.
But shape-shifting robots, which are controlled by magnetic fields, can dynamically squish, bend, or elongate their entire bodies.
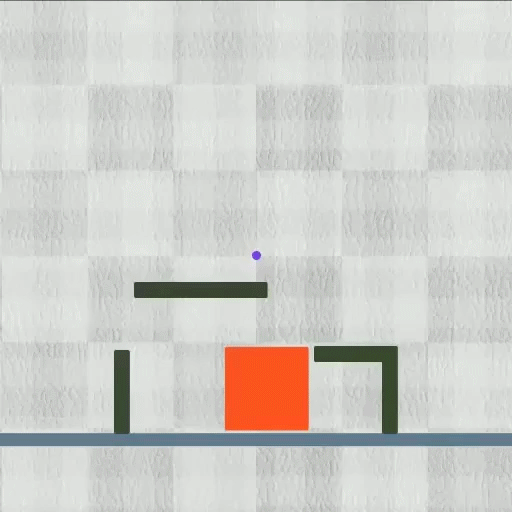
Image: Courtesy of the researchers
“Such a robot could have thousands of small pieces of muscle to control, so it is very hard to learn in a traditional way,” says Chen.
To solve this problem, he and his collaborators had to think about it differently. Rather than moving each tiny muscle individually, their reinforcement learning algorithm begins by learning to control groups of adjacent muscles that work together.
Then, after the algorithm has explored the space of possible actions by focusing on groups of muscles, it drills down into finer detail to optimize the policy, or action plan, it has learned. In this way, the control algorithm follows a coarse-to-fine methodology.
“Coarse-to-fine means that when you take a random action, that random action is likely to make a difference. The change in the outcome is likely very significant because you coarsely control several muscles at the same time,” Sitzmann says.
To enable this, the researchers treat a robot’s action space, or how it can move in a certain area, like an image.
Their machine-learning model uses images of the robot’s environment to generate a 2D action space, which includes the robot and the area around it. They simulate robot motion using what is known as the material-point-method, where the action space is covered by points, like image pixels, and overlayed with a grid.
The same way nearby pixels in an image are related (like the pixels that form a tree in a photo), they built their algorithm to understand that nearby action points have stronger correlations. Points around the robot’s “shoulder” will move similarly when it changes shape, while points on the robot’s “leg” will also move similarly, but in a different way than those on the “shoulder.”
In addition, the researchers use the same machine-learning model to look at the environment and predict the actions the robot should take, which makes it more efficient.
Building a simulator
After developing this approach, the researchers needed a way to test it, so they created a simulation environment called DittoGym.
DittoGym features eight tasks that evaluate a reconfigurable robot’s ability to dynamically change shape. In one, the robot must elongate and curve its body so it can weave around obstacles to reach a target point. In another, it must change its shape to mimic letters of the alphabet.
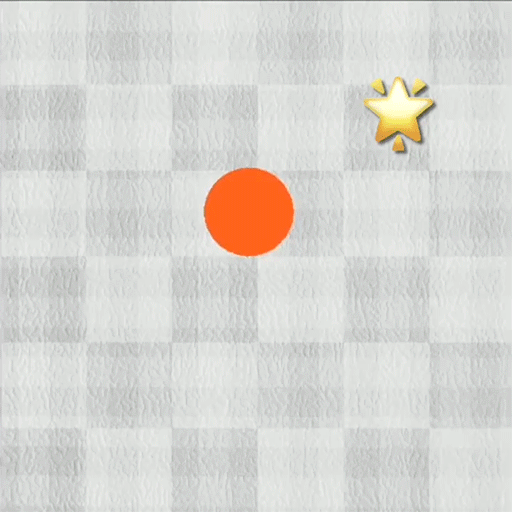
Image: Courtesy of the researchers
“Our task selection in DittoGym follows both generic reinforcement learning benchmark design principles and the specific needs of reconfigurable robots. Each task is designed to represent certain properties that we deem important, such as the capability to navigate through long-horizon explorations, the ability to analyze the environment, and interact with external objects,” Huang says. “We believe they together can give users a comprehensive understanding of the flexibility of reconfigurable robots and the effectiveness of our reinforcement learning scheme.”
Their algorithm outperformed baseline methods and was the only technique suitable for completing multistage tasks that required several shape changes.
“We have a stronger correlation between action points that are closer to each other, and I think that is key to making this work so well,” says Chen.
While it may be many years before shape-shifting robots are deployed in the real world, Chen and his collaborators hope their work inspires other scientists not only to study reconfigurable soft robots but also to think about leveraging 2D action spaces for other complex control problems.
What is AlphaFold 3? The AI Model Poised to Transform Biology
AlphaFold 3 is an AI model developed through a collaboration between Google DeepMind and Isomorphic Labs. This groundbreaking technology, which has garnered a lot of attention over the past couple of days as deserved, has achieved an unprecedented capability – accurately predicting the structure and interactions…
Hades II Cover Story, Xbox Studio Closures, And Animal Well Review | GI Show
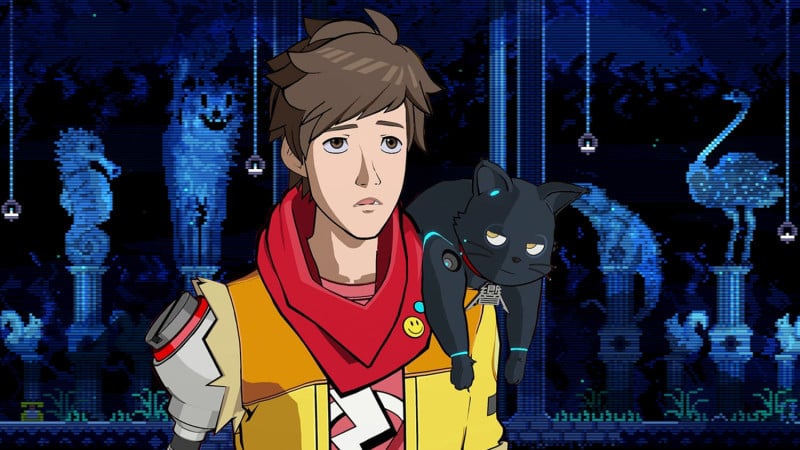
In this week’s episode of The Game Informer Show, we discuss our Hades II cover story trip to Supergiant Games in San Francisco and Xbox shutting down four game studios, including Prey, Dishonored, and Redfall developer Arkane Austin, alongside Tango Gameworks, the team behind The Evil Within series and last year’s critically-acclaimed Hi-Fi Rush. Afterward, we chat about Shuntaro Furukawa, President of Nintendo, revealing the company will reveal details about the Nintendo Switch’s successor within this fiscal year via a post on X. Before diving into our positive reviews of ’90s-survival-horror Crow Country and Little Kitty, Big City, special guest Charlie Wacholz joins us to chat about his Animal Well review, in which he calls Bigmode’s first published title “a masterclass in environmental design.”
Watch the Video Version:
[embedded content]
Follow us on social media: Alex Van Aken (@itsVanAken), Marcus Stewart (@MarcusStewart7), Wesley LeBlanc (@LeBlancWes), Charlie Wacholz (@chas_mke)
The Game Informer Show is a weekly gaming podcast covering the latest video game news, industry topics, exclusive reveals, and reviews. Join host Alex Van Aken every Thursday to chat about your favorite games – past and present – with Game Informer staff, developers, and special guests from around the industry. Listen on Apple Podcasts, Spotify, or your favorite podcast app.
The Game Informer Show – Podcast Timestamps:
00:00:00 – Intro
00:08:02 – Cover Story: Hades II
00:34:49 – Xbox Shutters Four Studios
00:53:49 – Nintendo Switch Successor Announcement
01:03:12 – Animal Well Review
01:28:21 – Little Kitty, Big City Review
01:35:00 – Crow Country
01:41:45 – Housekeeping
RED’s V-RAPTOR [X] Showcase and New Broadcast Solutions at NAB 2024 – Videoguys
![RED’s V-RAPTOR [X] Showcase and New Broadcast Solutions at NAB 2024 – Videoguys RED’s V-RAPTOR [X] Showcase and New Broadcast Solutions at NAB 2024 – Videoguys](https://videoguys.com/cdn/shop/articles/rednab2024.png?v=1715269144)
At NAB 2024, RED unveiled its cutting-edge V-RAPTOR [X] camera systems alongside groundbreaking Broadcast Solutions. Discover the latest innovations showcased at this year’s National Association of Broadcasters (NAB) Show.
Overview of RED’s Presence at NAB 2024:
At the NAB Show from April 13-18, RED introduced its revolutionary V-RAPTOR [X] and V-RAPTOR XL [X], marking a milestone as the premiere Large Format global shutter cinema camera. RED’s display centered around the Global Vision suite, featuring tools like Extended Highlights and Phantom Track tailored for both virtual and live production needs.
Focus on Broadcast Solutions:
RED’s debut of all-new broadcast technologies highlighted advancements geared towards enhancing cinematic imagery in broadcast and live event settings. Notably, the RED CINE-BROADCAST MODULE was unveiled to enable seamless integration with various V-RAPTOR camera systems.
Features of the RED CINE-BROADCAST MODULE:
This innovative module supports live broadcast capabilities with high-quality 4K 60P (HDR/SDR) output via 12G-SDI, along with IP-broadcast readiness utilizing SMPTE ST 2110 (TR-08) standards.
Advanced Workflow Enhancements:
Broadcasters can leverage RED Connect for expanded functionalities, including slow-motion, AI/ML integration, and live to headset experiences using 8K 120FPS R3Ds.
Introducing Broadcast Color Pipeline:
RED introduced the Broadcast Color pipeline for DSMC3 lineup, enabling real-time color adjustments and multi-camera color matching for broadcast and streaming applications.
RED’s presence at NAB 2024 was marked by groundbreaking advancements in camera technology and broadcast solutions. From the V-RAPTOR [X] launch to the unveiling of the RED CINE-BROADCAST MODULE and Broadcast Color pipeline, RED continues to push the boundaries of innovation in the industry.
[embedded content]
Jammable Review: How Good Are These AI Song Covers?
The world of music is constantly evolving, and with advancements in artificial intelligence, the possibilities for creative expression are endless. Jammable is a platform I recently came across that revolutionizes how we create music covers. Formerly known as Voicify AI, Jammable is an AI song cover…
Anand Sahay, CEO & Executive Director, Xebia – Interview Series
Anand Sahay is the CEO & Executive Director at Xebia, prior to joining Xebia, Sahay was a vice president at Interglobe and a general manager at HCL Technologies. He began his career as a software programmer at Tata Consultancy Services. Xebia is a pioneering and proven authority…