The war in Ukraine is based on two things – drones and artillery. Yes, other types of weapons…
What is Azure Service Fabric? Overview, Benefits and Features – Technology Org
What is Azure Service Fabric? Azure Service Fabric is a platform that enables developers to build, deploy, and…
Rocket Arena Will Shut Down In March
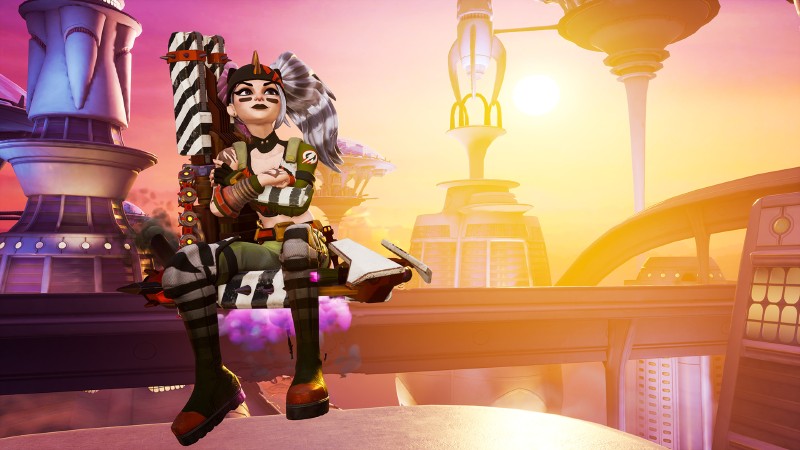
Rocket Arena, the multiplayer rocket-powered shooter, developed by First Strike Games as an EA Originals title, was removed from sale last month without warning or a word on why, as reported by RockPaperShotgun. Now, roughly a month later, First Strike Games has announced Rocket Arena will be sunset and its servers shut down in March.
More specifically, Rocket Arena will come to an end at Noon PT/3 p.m. ET on March 21, 2024. As for why, the team doesn’t really address that in its announcement but presumably, it has lost the player count needed to sustain its live-service, multiplayer model.
“It’s with a heavy heart from all of us at FSG and EA to inform you that Rocket Arena will be sunset and the servers shut down on [March 21, 2024],” the announcement reads. “We can’t thank you all enough for playing Rocket Arena and supporting us during this journey. We’re thrilled that people from all around the world got to enjoy Rocket Arena for three and a half years since it launched in July of 2020.
“Since then, there’s been a ton of Knockouts, Megablasts, Rocketball goals, Mega Rockets captured, and coins collected. We’ve been consistently blown away by the kindness, care, and camaraderie that’s been shown from the Rocket Arena community.”
The announcement continued, thanking the community for its great input over the years, before revealing the “Sunset Community Events.” You can check out the full list of events and content changes ahead of the game’s shutdown here, but it includes content from early Rocket Arena seasons and the removal of paid bundles in the store.
Rocket League first hit PlayStation 4, Xbox One, and PC on July 14, 2020. For our thoughts on the game, read Game Informer’s Rocket Arena review.
Are you going to hop back into Rocket Arena before its shutdown next year? Let us know in the comments below!
Platinum Games Co-Founder Hideki Kamiya Reveals Why He Left The Studio
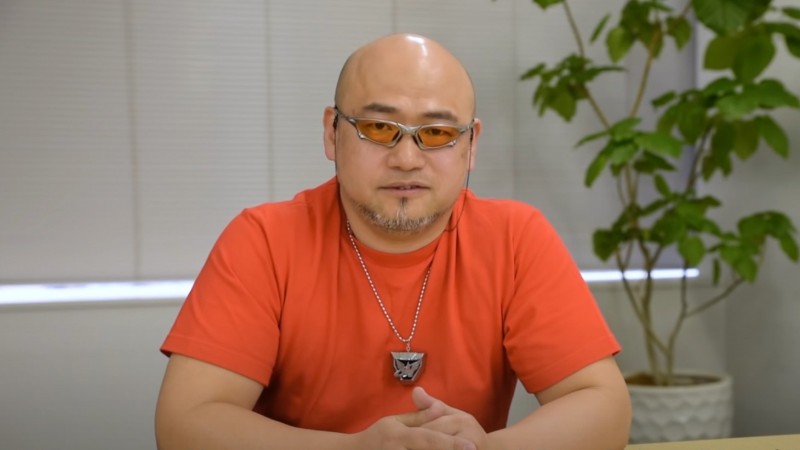
More than two months after leaving Platinum Games, co-founder and The Wonderful 101 and Bayonetta director Hideki Kamiya has revealed why he left the studio. In a new interview with IGN, Kamiya told the publication he decided to leave “because I felt that the direction the company was heading in was different from my beliefs as a developer.”
“Without that element of trust, I couldn’t continue working there, and so I left, so that I could continue working in what I consider to be the right way,” Kamiya said. “I don’t think of games as products, but rather as works of art. I want to put my artistry into games and deliver games that could only be made by Hideki Kamiya, so that players can enjoy Hideki Kamiya games exactly as they are.
“I decided to leave the company and forge my own path, to continue making games that reflect the developers who made them.”
[embedded content]
Kamiya announced in September that he was leaving the company. His final day was October 12, and that same day, he revealed he had started a YouTube Channel. Throughout his final month at Platinum, his departure, and creation of the Hideki Kamiya Channel, Kamiya hadn’t revealed why he left the studio, leaving fans questioning the decision. Thanks to this new interview with IGN, we now know it was about creative direction.
Elsehwere in the interview, Kamiya said he had many deep discussions with Platinum president Atsushi Inaba about his potential departure, deciding to leave only after the two agreed.
“Inaba also had his own set of beliefs as the head of the company,” Kamiya told IGN. “Although his beliefs differed from my own, we talked it out thoroughly, so we were both satisfied with the conclusion we reached.”
Kamiya said the reaction from the development teams at Platinum was one of sadness, with some even crying in his office while talking to him about it. Despite the sadness, Kamiya said he doesn’t regret his decision to quit.
[embedded content]
For more about Kamiya’s departure, his future, and his ideas around game development, be sure to read IGN’s full interview here.
Before co-founding Platinum in February of 2006, Kamiya worked on Capcom’s Resident Evil series, helping plan the first game and directing its sequel, as well as its Devil May Cry series. He also directed the first Viewtiful Joe game, and then Okami in 2006 at Capcom’s Clover Studio. Capcom closed Clover later that year but Platinum had already been somewhat founded under the name Seeds, Inc. It was later renamed Platinum Games and the rest was history.
Kamiya departs from Platinum more than 17 years after he founded it alongside Shinji Mikami, who is no longer there and departed from Tango Gameworks earlier this year, and Atsushi Inaba, who is the studio’s CEO.
While waiting to learn what Kamiya is up to next, read Game Informer’s review of Bayonetta and then check out our thoughts on The Wonderful 101. Read Game Informer’s Bayonetta 3 review after that and then read our review of Bayonetta Origins: Cereza and the Lost Demon.
[Source: IGN]
What do you hope Kamiya does next? Let us know in the comments below!
Your Ultimate Guide to Magento 2 Migration: Prepare for Success in 2024 – Technology Org
One of the many things that the eCommerce world demands is staying updated with the latest trends and…
Chance Twists Ordered Carbon Nanotubes Into ‘Tornado Films’ – Technology Org
Rice University study uncovers new ways to make ordered wafer-scale chiral carbon nanotube architectures. Chiral materials interact with…
Particle Physicists Put Forward Research Priorities for Coming Decade – Technology Org
A panel of the nation’s top particle physicists, chaired by University of California, Berkeley, theoretician Hitoshi Murayama, has issued…
When Is an Aurora Not an Aurora? – Technology Org
The shimmering green, red and purple curtains of the northern and southern lights — the auroras — may…
Carbon Nanotubes Have Progressed Towards Energy and Health Applications, but Misconceptions Remain – Technology Org
The increasing use of carbon nanotubes (CNTs) — and a proposal in the European Union to ban the entire class…
Computational model captures the elusive transition states of chemical reactions
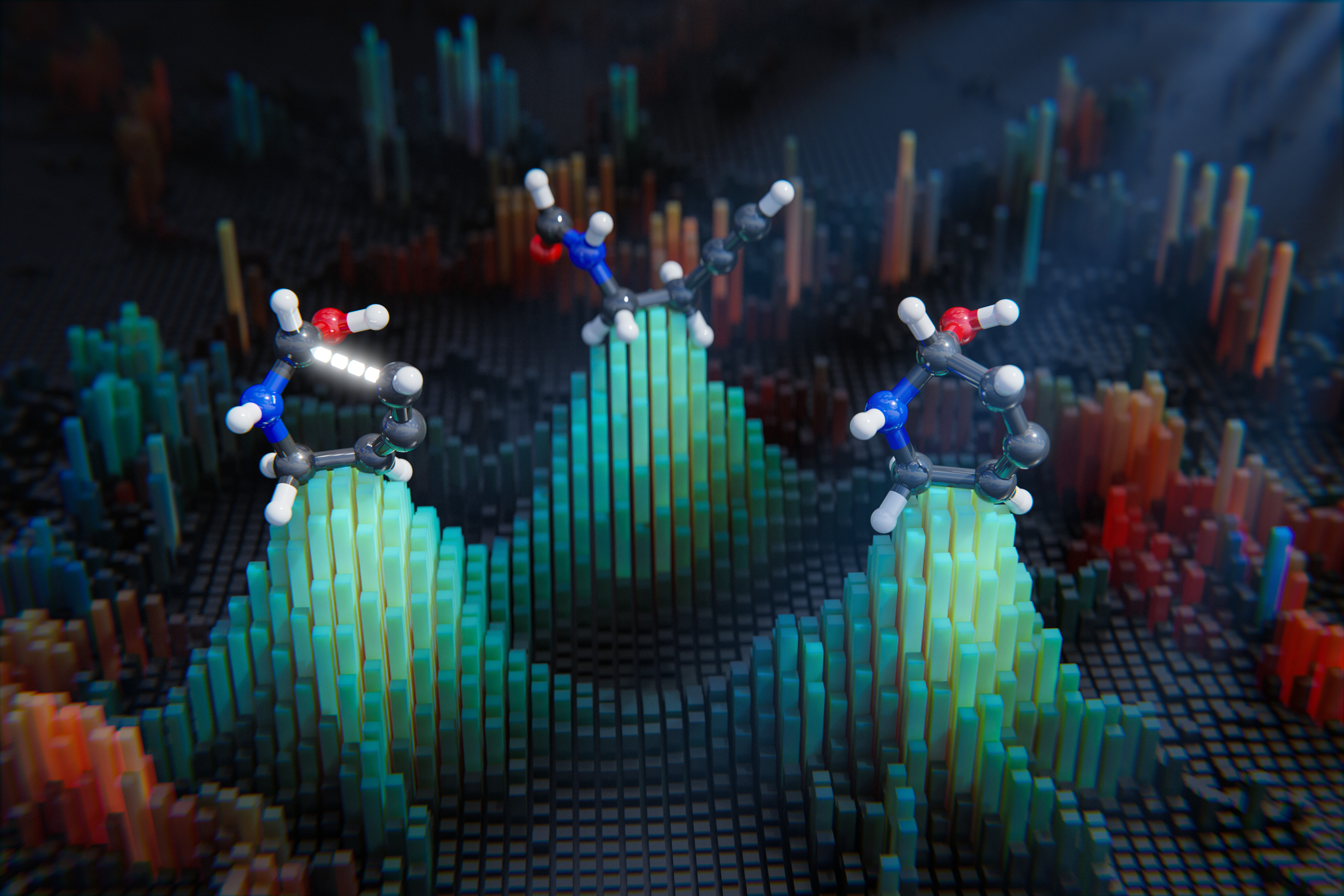
During a chemical reaction, molecules gain energy until they reach what’s known as the transition state — a point of no return from which the reaction must proceed. This state is so fleeting that it’s nearly impossible to observe it experimentally.
The structures of these transition states can be calculated using techniques based on quantum chemistry, but that process is extremely time-consuming. A team of MIT researchers has now developed an alternative approach, based on machine learning, that can calculate these structures much more quickly — within a few seconds.
Their new model could be used to help chemists design new reactions and catalysts to generate useful products like fuels or drugs, or to model naturally occurring chemical reactions such as those that might have helped to drive the evolution of life on Earth.
“Knowing that transition state structure is really important as a starting point for thinking about designing catalysts or understanding how natural systems enact certain transformations,” says Heather Kulik, an associate professor of chemistry and chemical engineering at MIT, and the senior author of the study.
Chenru Duan PhD ’22 is the lead author of a paper describing the work, which appears today in Nature Computational Science. Cornell University graduate student Yuanqi Du and MIT graduate student Haojun Jia are also authors of the paper.
Fleeting transitions
For any given chemical reaction to occur, it must go through a transition state, which takes place when it reaches the energy threshold needed for the reaction to proceed. The probability of any chemical reaction occurring is partly determined by how likely it is that the transition state will form.
“The transition state helps to determine the likelihood of a chemical transformation happening. If we have a lot of something that we don’t want, like carbon dioxide, and we’d like to convert it to a useful fuel like methanol, the transition state and how favorable that is determines how likely we are to get from the reactant to the product,” Kulik says.
Chemists can calculate transition states using a quantum chemistry method known as density functional theory. However, this method requires a huge amount of computing power and can take many hours or even days to calculate just one transition state.
Recently, some researchers have tried to use machine-learning models to discover transition state structures. However, models developed so far require considering two reactants as a single entity in which the reactants maintain the same orientation with respect to each other. Any other possible orientations must be modeled as separate reactions, which adds to the computation time.
“If the reactant molecules are rotated, then in principle, before and after this rotation they can still undergo the same chemical reaction. But in the traditional machine-learning approach, the model will see these as two different reactions. That makes the machine-learning training much harder, as well as less accurate,” Duan says.
The MIT team developed a new computational approach that allowed them to represent two reactants in any arbitrary orientation with respect to each other, using a type of model known as a diffusion model, which can learn which types of processes are most likely to generate a particular outcome. As training data for their model, the researchers used structures of reactants, products, and transition states that had been calculated using quantum computation methods, for 9,000 different chemical reactions.
“Once the model learns the underlying distribution of how these three structures coexist, we can give it new reactants and products, and it will try to generate a transition state structure that pairs with those reactants and products,” Duan says.
The researchers tested their model on about 1,000 reactions that it hadn’t seen before, asking it to generate 40 possible solutions for each transition state. They then used a “confidence model” to predict which states were the most likely to occur. These solutions were accurate to within 0.08 angstroms (one hundred-millionth of a centimeter) when compared to transition state structures generated using quantum techniques. The entire computational process takes just a few seconds for each reaction.
“You can imagine that really scales to thinking about generating thousands of transition states in the time that it would normally take you to generate just a handful with the conventional method,” Kulik says.
Modeling reactions
Although the researchers trained their model primarily on reactions involving compounds with a relatively small number of atoms — up to 23 atoms for the entire system — they found that it could also make accurate predictions for reactions involving larger molecules.
“Even if you look at bigger systems or systems catalyzed by enzymes, you’re getting pretty good coverage of the different types of ways that atoms are most likely to rearrange,” Kulik says.
The researchers now plan to expand their model to incorporate other components such as catalysts, which could help them investigate how much a particular catalyst would speed up a reaction. This could be useful for developing new processes for generating pharmaceuticals, fuels, or other useful compounds, especially when the synthesis involves many chemical steps.
“Traditionally all of these calculations are performed with quantum chemistry, and now we’re able to replace the quantum chemistry part with this fast generative model,” Duan says.
Another potential application for this kind of model is exploring the interactions that might occur between gases found on other planets, or to model the simple reactions that may have occurred during the early evolution of life on Earth, the researchers say.
The new method represents “a significant step forward in predicting chemical reactivity,” says Jan Halborg Jensen, a professor of chemistry at the University of Copenhagen, who was not involved in the research.
“Finding the transition state of a reaction and the associated barrier is the key step in predicting chemical reactivity, but also the one of the hardest tasks to automate,” he says. “This problem is holding back many important fields such as computational catalyst and reaction discovery, and this is the first paper I have seen that could remove this bottleneck.”
The research was funded by the U.S. Office of Naval Research and the National Science Foundation.