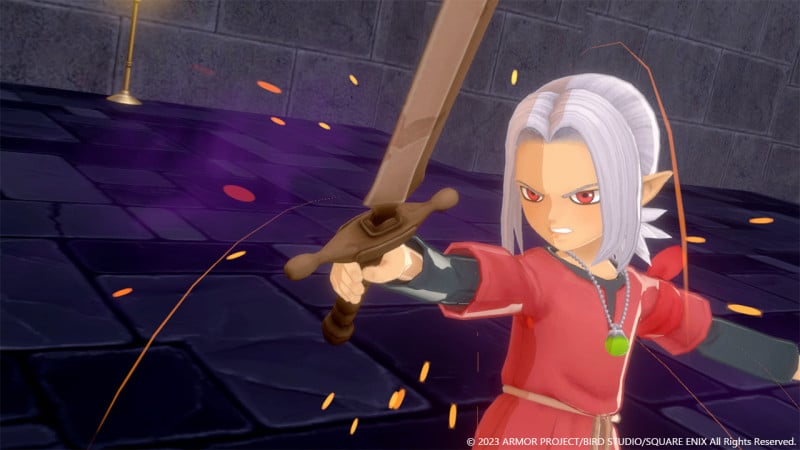
Dragon Quest possesses so much history that any new game carries a degree of raised expectation. Dragon Quest Monsters: The Dark Prince delivers many of the conventions I’ve come to expect from the series: the vibrant opening song, the charismatic Slime, and the emotional storytelling I already associate with the franchise. But this game goes beyond those well-treaded territories, offering an intelligent and elegant yet simple approach to combat and dungeon design that makes it a solid spin-off experience.
In The Dark Prince, you play as Psaro, a half-human, half-monster boy who becomes a powerful monster wrangler because of a curse that makes fighting them with his own hands impossible. Wrangling is all about capturing monsters and controlling them during turn-based battles. As I progressed throughout the boy’s journey, I found stronger creatures to add to my roster. The game also has an online mode that lets you fight other players, which is a good way to test different group compositions. In my case, it took so long to find a match that my time was better spent adventuring solo.
Synthesizing new monsters by fusing two parent creatures is the best method to obtain a better team, and this system makes all your effort in previously maximizing weaker monsters worth it. Whenever you create a new monster, it’s possible to keep some of the skill points spent in the creatures you’re fusing. Through this system, I crafted some extremely powerful monsters, surpassing their regular versions found in the wild. This system pushes you towards excessive grinding, though. Whenever you fuse a new creature, it comes at level 1, regardless of its parents’ levels. In the final sections of the game, fusing a new monster at the wrong moment means spending a lot of time leveling up before getting back on track and trying to defeat a boss.
With the vast number of possible monster combinations you can create in The Dark Prince, I was surprised by how streamlined combat is. The game allows you to set up tactics that define whether a party member will focus on attacking enemies or healing other party members, for example. At the same time, it’s possible to order specific actions for each monster. However, outside of boss fights, engaging more strategically in battles rarely felt necessary. The system waters down so much of each encounter that I usually entered automatic mode and let the A.I. do the thinking.
As the boy works on his craft, we learn about Psaro’s past and his journey alongside his friends, Rose and Toilen, to become strong enough to challenge his father. This is a classic, almost too familiar, premise, but even with the absence of heavily foreshadowed surprises or plot twists, The Dark Prince captivated me, making for a cozy adventure with the charm of an old-fashioned fairy tale.
The game presents the same slow-paced introduction other Dragon Quest games have, making the first few hours a slog. However, I became slowly entangled in the story. Initially, I was progressing only to unlock new monsters, but I realized I was as excited about learning more about Psaro’s tale as I was about finding new creatures. Unfortunately, very few situations offer even a glimpse of what he’s thinking, and it never gave me a chance to understand the reasoning behind his acts better. In this aspect, the game’s respect for its roots hinders its capacity to develop an intriguing character with no option besides nodding or saying yes or no.
While perfectly capable as a standalone title, The Dark Prince is directly connected to Dragon Quest IV. It gives us a chance to learn more about Psaro, a crucial figure in the older title, and also to look over some events related to the previous game from a different perspective.
[embedded content]
Psaro’s journey takes us through areas in Nadiria, a magical dimension with different regions called circles. Each circle splits into three tiers, with one final dungeon. Sadly, this structure makes for a repetitive and predictable pattern; after completing the first four circles, I knew exactly what to expect from every new region. These areas are made worse by noticeable dips in performance, as the framerate suffers considerably. While I could easily ignore these minor performance issues, the circles’ recursive design became more tiresome whenever I went for long gameplay sessions.
On the other hand, the dungeons are the most surprising element of each circle. They all share a similar structure: many floors, a traversal gimmick, and a teleport before the boss room. While they might feel as repetitive as the circles, the puzzles inside each dungeon make them fun and varied. The developers found a solid balance between difficulty and enjoyment when designing them. The Dark Prince veers more toward traditional dungeon design, with treadmills you need to activate to advance or ladders and holes in the ground to get to the top of the building. Though most dungeons are forgettable, they offer a refreshing intellectual experience even without leaning on any design extravaganza.
By rigidly following Dragon Quest traditions, we end up with flat, cartoonish characters who inhabit a repetitive, cyclical world. But The Dark Prince plays to its strengths to deliver a solid RPG experience with a cozy narrative seasoned by a long list of charismatic creatures and entertaining dungeons.