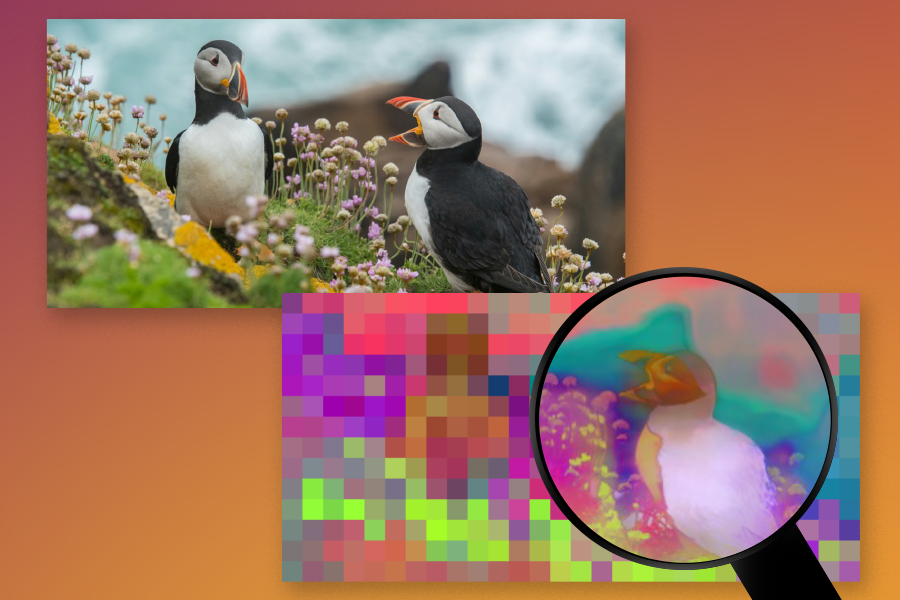
Imagine yourself glancing at a busy street for a few moments, then trying to sketch the scene you saw from memory. Most people could draw the rough positions of the major objects like cars, people, and crosswalks, but almost no one can draw every detail with pixel-perfect accuracy. The same is true for most modern computer vision algorithms: They are fantastic at capturing high-level details of a scene, but they lose fine-grained details as they process information.
Now, MIT researchers have created a system called “FeatUp” that lets algorithms capture all of the high- and low-level details of a scene at the same time — almost like Lasik eye surgery for computer vision.
When computers learn to “see” from looking at images and videos, they build up “ideas” of what’s in a scene through something called “features.” To create these features, deep networks and visual foundation models break down images into a grid of tiny squares and process these squares as a group to determine what’s going on in a photo. Each tiny square is usually made up of anywhere from 16 to 32 pixels, so the resolution of these algorithms is dramatically smaller than the images they work with. In trying to summarize and understand photos, algorithms lose a ton of pixel clarity.
The FeatUp algorithm can stop this loss of information and boost the resolution of any deep network without compromising on speed or quality. This allows researchers to quickly and easily improve the resolution of any new or existing algorithm. For example, imagine trying to interpret the predictions of a lung cancer detection algorithm with the goal of localizing the tumor. Applying FeatUp before interpreting the algorithm using a method like class activation maps (CAM) can yield a dramatically more detailed (16-32x) view of where the tumor might be located according to the model.
FeatUp not only helps practitioners understand their models, but also can improve a panoply of different tasks like object detection, semantic segmentation (assigning labels to pixels in an image with object labels), and depth estimation. It achieves this by providing more accurate, high-resolution features, which are crucial for building vision applications ranging from autonomous driving to medical imaging.
“The essence of all computer vision lies in these deep, intelligent features that emerge from the depths of deep learning architectures. The big challenge of modern algorithms is that they reduce large images to very small grids of ‘smart’ features, gaining intelligent insights but losing the finer details,” says Mark Hamilton, an MIT PhD student in electrical engineering and computer science, MIT Computer Science and Artificial Intelligence Laboratory (CSAIL) affiliate, and a co-lead author on a paper about the project. “FeatUp helps enable the best of both worlds: highly intelligent representations with the original image’s resolution. These high-resolution features significantly boost performance across a spectrum of computer vision tasks, from enhancing object detection and improving depth prediction to providing a deeper understanding of your network’s decision-making process through high-resolution analysis.”
Resolution renaissance
As these large AI models become more and more prevalent, there’s an increasing need to explain what they’re doing, what they’re looking at, and what they’re thinking.
But how exactly can FeatUp discover these fine-grained details? Curiously, the secret lies in wiggling and jiggling images.
In particular, FeatUp applies minor adjustments (like moving the image a few pixels to the left or right) and watches how an algorithm responds to these slight movements of the image. This results in hundreds of deep-feature maps that are all slightly different, which can be combined into a single crisp, high-resolution, set of deep features. “We imagine that some high-resolution features exist, and that when we wiggle them and blur them, they will match all of the original, lower-resolution features from the wiggled images. Our goal is to learn how to refine the low-resolution features into high-resolution features using this ‘game’ that lets us know how well we are doing,” says Hamilton. This methodology is analogous to how algorithms can create a 3D model from multiple 2D images by ensuring that the predicted 3D object matches all of the 2D photos used to create it. In FeatUp’s case, they predict a high-resolution feature map that’s consistent with all of the low-resolution feature maps formed by jittering the original image.
The team notes that standard tools available in PyTorch were insufficient for their needs, and introduced a new type of deep network layer in their quest for a speedy and efficient solution. Their custom layer, a special joint bilateral upsampling operation, was over 100 times more efficient than a naive implementation in PyTorch. The team also showed this new layer could improve a wide variety of different algorithms including semantic segmentation and depth prediction. This layer improved the network’s ability to process and understand high-resolution details, giving any algorithm that used it a substantial performance boost.
“Another application is something called small object retrieval, where our algorithm allows for precise localization of objects. For example, even in cluttered road scenes algorithms enriched with FeatUp can see tiny objects like traffic cones, reflectors, lights, and potholes where their low-resolution cousins fail. This demonstrates its capability to enhance coarse features into finely detailed signals,” says Stephanie Fu ’22, MNG ’23, a PhD student at the University of California at Berkeley and another co-lead author on the new FeatUp paper. “This is especially critical for time-sensitive tasks, like pinpointing a traffic sign on a cluttered expressway in a driverless car. This can not only improve the accuracy of such tasks by turning broad guesses into exact localizations, but might also make these systems more reliable, interpretable, and trustworthy.”
What next?
Regarding future aspirations, the team emphasizes FeatUp’s potential widespread adoption within the research community and beyond, akin to data augmentation practices. “The goal is to make this method a fundamental tool in deep learning, enriching models to perceive the world in greater detail without the computational inefficiency of traditional high-resolution processing,” says Fu.
“FeatUp represents a wonderful advance towards making visual representations really useful, by producing them at full image resolutions,” says Cornell University computer science professor Noah Snavely, who was not involved in the research. “Learned visual representations have become really good in the last few years, but they are almost always produced at very low resolution — you might put in a nice full-resolution photo, and get back a tiny, postage stamp-sized grid of features. That’s a problem if you want to use those features in applications that produce full-resolution outputs. FeatUp solves this problem in a creative way by combining classic ideas in super-resolution with modern learning approaches, leading to beautiful, high-resolution feature maps.”
“We hope this simple idea can have broad application. It provides high-resolution versions of image analytics that we’d thought before could only be low-resolution,” says senior author William T. Freeman, an MIT professor of electrical engineering and computer science professor and CSAIL member.
Lead authors Fu and Hamilton are accompanied by MIT PhD students Laura Brandt SM ’21 and Axel Feldmann SM ’21, as well as Zhoutong Zhang SM ’21, PhD ’22, all current or former affiliates of MIT CSAIL. Their research is supported, in part, by a National Science Foundation Graduate Research Fellowship, by the National Science Foundation and Office of the Director of National Intelligence, by the U.S. Air Force Research Laboratory, and by the U.S. Air Force Artificial Intelligence Accelerator. The group will present their work in May at the International Conference on Learning Representations.